Improving home insurance pricing with synthetic geolocation data
公司规模
1,000+
地区
- America
国家
- United States
产品
- Synthetic Geolocation Data
技术栈
- Synthetic Data Generation
- Data Modeling
- Public Databases
实施规模
- Enterprise-wide Deployment
影响指标
- Cost Savings
- Digital Expertise
- Productivity Improvements
技术
- 分析与建模 - 数据即服务
- 分析与建模 - 预测分析
- 应用基础设施与中间件 - 数据交换与集成
适用行业
- 金融与保险
适用功能
- 商业运营
- 质量保证
用例
- 监管合规监控
服务
- 数据科学服务
- 系统集成
关于客户
The customer is a large insurance company operating across the United States, providing home insurance to a diverse range of clients. The company faces the challenge of pricing insurance policies accurately due to the varying climate features and risk profiles of different regions. They are also bound by strict regulations such as CCPA and HIPPA, which prevent them from using personal data like customer addresses in their risk assessment models. This limitation has made it difficult for the company to accurately assess risk and set appropriate pricing for their insurance policies.
挑战
Home insurance pricing was a risky business for our client. The insurance company catered to homes across the United States in areas with vastly different climate features and risk profiles. CCPA and HIPPA forbade the data science team to use the customers’ personal data, such as their addresses, in their modeling, so they could not assess risk and reflect that in their pricing.
解决方案
The insurance company served modeling teams with synthetic geolocation data. The team could use synthetic home addresses to look up five climate features, such as fire and flood hazards, in public databases. The pricing model trained on synthetic data scored as good as the model trained on real data. Using synthetic home addresses eliminated the risk of re-identification and unlocked new insights. The team established a synthetization framework tailored to modeling based on privacy-risk classification and shortened time-to-data from 6 months to 3 days. The process kept 100% utility of the data, perfectly retaining the statistical dispersion of the original and providing an as-good-as real data alternative for training.
运营影响
数量效益
Case Study missing?
Start adding your own!
Register with your work email and create a new case study profile for your business.
相关案例.
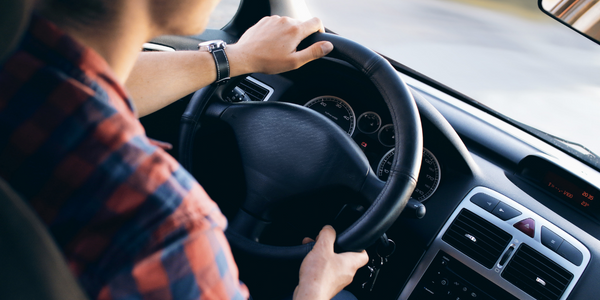
Case Study
Real-time In-vehicle Monitoring
The telematic solution provides this vital premium-adjusting information. The solution also helps detect and deter vehicle or trailer theft – as soon as a theft occurs, monitoring personnel can alert the appropriate authorities, providing an exact location.“With more and more insurance companies and major fleet operators interested in monitoring driver behaviour on the grounds of road safety, efficient logistics and costs, the market for this type of device and associated e-business services is growing rapidly within Italy and the rest of Europe,” says Franco.“The insurance companies are especially interested in the pay-per-use and pay-as-you-drive applications while other organisations employ the technology for road user charging.”“One million vehicles in Italy currently carry such devices and forecasts indicate that the European market will increase tenfold by 2014.However, for our technology to work effectively, we needed a highly reliable wireless data network to carry the information between the vehicles and monitoring stations.”
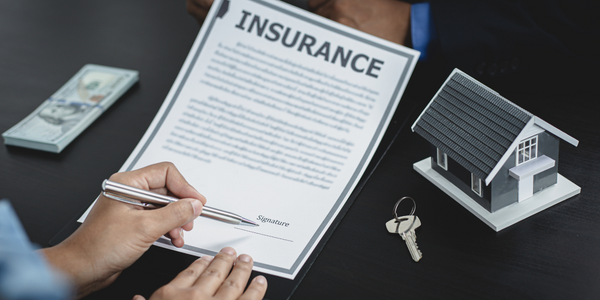
Case Study
Safety First with Folksam
The competitiveness of the car insurance market is driving UBI growth as a means for insurance companies to differentiate their customer propositions as well as improving operational efficiency. An insurance model - usage-based insurance ("UBI") - offers possibilities for insurers to do more efficient market segmentation and accurate risk assessment and pricing. Insurers require an IoT solution for the purpose of data collection and performance analysis
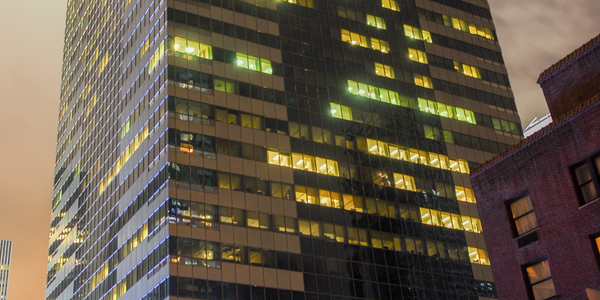
Case Study
Smooth Transition to Energy Savings
The building was equipped with four end-of-life Trane water cooled chillers, located in the basement. Johnson Controls installed four York water cooled centrifugal chillers with unit mounted variable speed drives and a total installed cooling capacity of 6,8 MW. Each chiller has a capacity of 1,6 MW (variable to 1.9MW depending upon condenser water temperatures). Johnson Controls needed to design the equipment in such way that it would fit the dimensional constraints of the existing plant area and plant access route but also the specific performance requirements of the client. Morgan Stanley required the chiller plant to match the building load profile, turn down to match the low load requirement when needed and provide an improvement in the Energy Efficiency Ratio across the entire operating range. Other requirements were a reduction in the chiller noise level to improve the working environment in the plant room and a wide operating envelope coupled with intelligent controls to allow possible variation in both flow rate and temperature. The latter was needed to leverage increased capacity from a reduced number of machines during the different installation phases and allow future enhancement to a variable primary flow system.
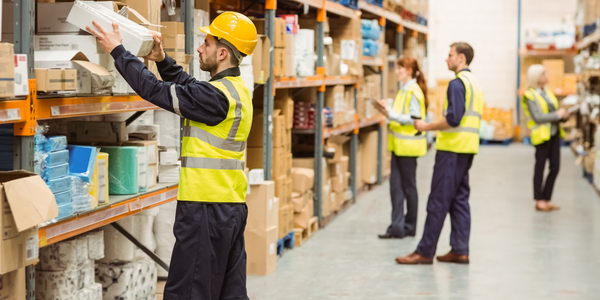
Case Study
Automated Pallet Labeling Solution for SPR Packaging
SPR Packaging, an American supplier of packaging solutions, was in search of an automated pallet labeling solution that could meet their immediate and future needs. They aimed to equip their lines with automatic printer applicators, but also required a solution that could interface with their accounting software. The challenge was to find a system that could read a 2D code on pallets at the stretch wrapper, track the pallet, and flag any pallets with unread barcodes for inspection. The pallets could be single or double stacked, and the system needed to be able to differentiate between the two. SPR Packaging sought a system integrator with extensive experience in advanced printing and tracking solutions to provide a complete traceability system.
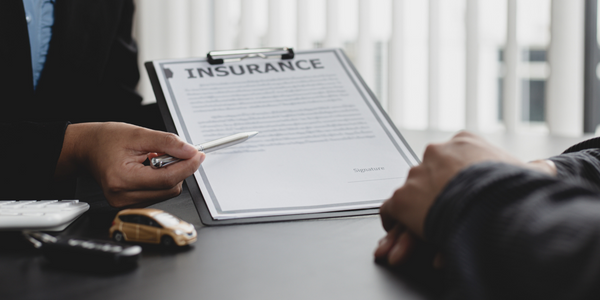
Case Study
Transforming insurance pricing while improving driver safety
The Internet of Things (IoT) is revolutionizing the car insurance industry on a scale not seen since the introduction of the car itself. For decades, premiums have been calculated using proxy-based risk assessment models and historical data. Today, a growing number of innovative companies such as Quebec-based Industrielle Alliance are moving to usage-based insurance (UBI) models, driven by the advancement of telematics technologies and smart tracking devices.

Case Study
MasterCard Improves Customer Experience Through Self-Service Data Prep
Derek Madison, Leader of Business Financial Support at MasterCard, oversees the validation of transactions and cash between two systems, whether they’re MasterCard owned or not. He was charged with identifying new ways to increase efficiency and improve MasterCard processes. At the outset, the 13-person team had to manually reconcile system interfaces using reports that resided on the company’s mainframe. Their first order of business each day was to print 20-30 individual, multi-page reports. Using a ruler to keep their place within each report, they would then hand-key the relevant data, line by line, into Excel for validation. “We’re talking about a task that took 40-80 hours each week,” recalls Madison, “As a growing company with rapidly expanding product offerings, we had to find a better way to prepare this data for analysis.”