Product
- Gathr
Tech Stack
- AWS IoT Gateway
- Apache Spark
Impact Metrics
- Cost Savings
- Customer Satisfaction
Technology Category
- Analytics & Modeling - Machine Learning
- Analytics & Modeling - Real Time Analytics
Applicable Industries
- Automotive
Applicable Functions
- Business Operation
- Sales & Marketing
Use Cases
- Predictive Maintenance
- Usage-Based Insurance
- Vehicle Telematics
Services
- Data Science Services
About The Customer
The customer is a leading auto insurance provider. To keep up with the new digital consumer and remain competitive, the company is increasingly investing in connected car solutions. The company uses a telematics device to capture and transmit vehicle performance, usage, and driver behavior data from various sensors in the car. The company chose Gathr to ingest, transform, enrich, analyze and store automotive telematics data in real-time to build an end-to-end analytics application for driver profiling & individual risk assessment, and subsequently offer dynamic, usage-based, plans to its customers.
The Challenge
The auto insurance industry is increasingly investing in connected car solutions to offer simplified, transparent, and flexible products and pricing options. Usage-based insurance is a voluntary, behavior-based insurance program that uses analytics to create highly personalized and dynamic plans based not only on the driver’s age and other demographics, but also accounts for the driver’s behavior, risks related to a vehicle, and external factors such as driving conditions and weather. A leading auto insurance provider chose Gathr to ingest, transform, enrich, analyze and store automotive telematics data in real-time to build an end-to-end analytics application for driver profiling & individual risk assessment, and subsequently offer dynamic, usage-based, plans to its customers.
The Solution
The solution involves real-time ingestion of telematics and sensor data using an AWS IoT gateway. The device captures data points such as driver behavior, vehicle sensor data, and usage data. In-memory data transformation, data blending, and data enrichment are performed as driver behavior, usage, and vehicle data arrives. The ingestion and enrichment stages provide a rich array of key attributes needed for the predictive machine learning models running on Apache Spark. These stages assess and predict individual risk scores. Classifying drivers as safe or risky and quantifying risk scores are based on current driving behavior, historical behavior, and supplemental data flows such as usage data, geographic location, vehicle type, vehicle performance, and third-party data. The application creates alerts to flag risks based on altered behavior patterns as well as anomalies in vehicle performance.
Operational Impact
Quantitative Benefit
Case Study missing?
Start adding your own!
Register with your work email and create a new case study profile for your business.
Related Case Studies.
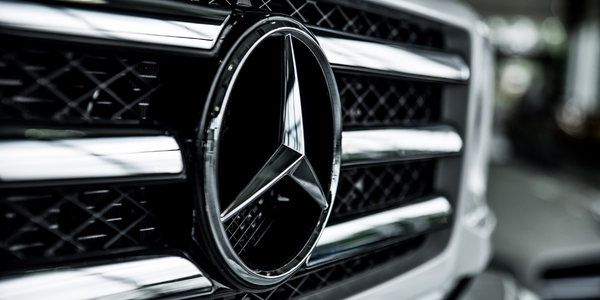
Case Study
Integral Plant Maintenance
Mercedes-Benz and his partner GAZ chose Siemens to be its maintenance partner at a new engine plant in Yaroslavl, Russia. The new plant offers a capacity to manufacture diesel engines for the Russian market, for locally produced Sprinter Classic. In addition to engines for the local market, the Yaroslavl plant will also produce spare parts. Mercedes-Benz Russia and his partner needed a service partner in order to ensure the operation of these lines in a maintenance partnership arrangement. The challenges included coordinating the entire maintenance management operation, in particular inspections, corrective and predictive maintenance activities, and the optimizing spare parts management. Siemens developed a customized maintenance solution that includes all electronic and mechanical maintenance activities (Integral Plant Maintenance).
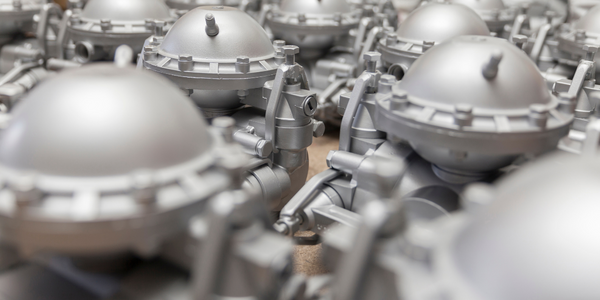
Case Study
Monitoring of Pressure Pumps in Automotive Industry
A large German/American producer of auto parts uses high-pressure pumps to deburr machined parts as a part of its production and quality check process. They decided to monitor these pumps to make sure they work properly and that they can see any indications leading to a potential failure before it affects their process.