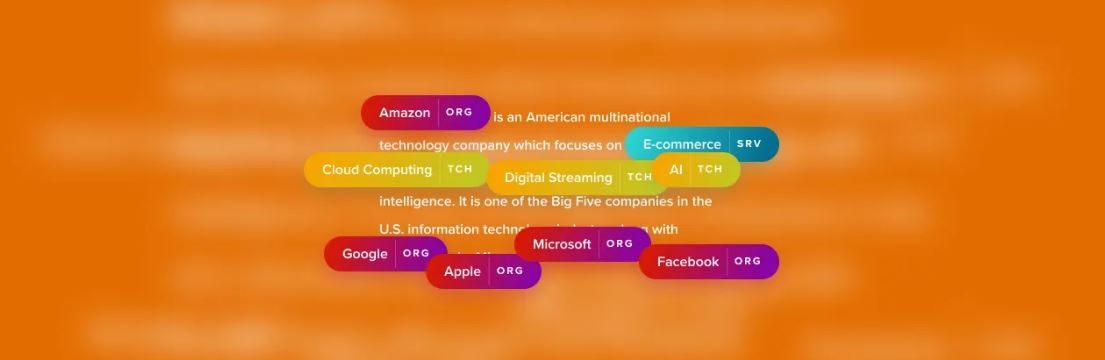
Not disclosed
The client is a leading global financial consulting company that supports financial advisors, consultants, and institutional investors.
The client, operating in the financial sector, is working with large collections of client meeting notes in order to search for certain information, then organize and consolidate it into easy-to-read interactive sets so it could be used for different business purposes.
So, they needed to search, find, and process texts quickly, and obtain actual insights from the data across the notes, in order to understand their (potential) customers, and identify market trends and risks for further informed business decisions by tagging documents with labels.
To enable fast searches through the text that would reveal actual valuable information, out-of-the-box and custom approaches were compared:
- An out-of-the-box cloud solution that leverages the AWS Comprehend service.
- Custom solution based on Topic Modeling using LDA and Named Entity Recognition (NER) to tag documents with labels in order to enable a search through raw unstructured texts.
The use of Topic Modeling helps to determine the list of topics discussed in an entire collection of meeting notes and that reveals the presence of one or more topics in each document.
Using custom NER solutions, based on a deep learning model, they can detect both default and custom entities in real-time.
Case Study missing?
Start adding your own!
Register with your work email and create a new case study profile for your business.