公司规模
200-1,000
地区
- Asia
- Pacific
国家
- Worldwide
产品
- Sift's machine learning system
技术栈
- Machine Learning
- REST API
实施规模
- Enterprise-wide Deployment
影响指标
- Brand Awareness
- Customer Satisfaction
技术
- 分析与建模 - 机器学习
适用功能
- 商业运营
用例
- 欺诈识别
服务
- 数据科学服务
关于客户
Travelmob, acquired by HomeAway, is a platform created to help global travelers find unique places to stay across Asia Pacific, from Bangkok to Melbourne. Hosts list their rooms and properties, and guests use the Travelmob site or mobile app to book them. The company's customer experience is key to its business model, as it directly impacts the brand experience, repeat business, new users, and continued growth. However, the company was facing challenges with fake listings and credit card fraud, which were negatively impacting the customer experience and resulting in costly chargebacks.
挑战
Travelmob, a social marketplace for travellers, was facing a growing trend of fake listings on its site. Bad users were posing as legitimate hosts, posting photos of properties they didn’t own, and trying to con unsuspecting guests into making their payment offsite. This was negatively impacting the customer experience and the company's brand image. Additionally, the company was also dealing with credit card fraud that was resulting in costly chargebacks. Initially, Travelmob began by manually reviewing new listings and booking requests, but this approach was not scalable and fraud was slipping through the cracks. Building dedicated internal tools for fighting fraud would require time and resources that they couldn’t spare, and anything they created internally couldn’t adequately address the complexity of fraud.
解决方案
Travelmob decided to implement Sift's machine-learning fraud solution. After perusing Sift’s easy-to-use REST API, it only took a few hours for the Travelmob team to get the system up and running, and they were fully integrated within a week. Travelmob used Sift Scores to identify high-risk bookings that required manual review. They also used advanced tools and rich insights in the Sift Console, like Network Visualizations, to connect the dots between different users and locations and make smart decisions about who to block. Initially, Travelmob used Sift to catch fake listings, but the experience was so successful that they also applied the machine learning solution to their credit card fraud problem.
运营影响
Case Study missing?
Start adding your own!
Register with your work email and create a new case study profile for your business.
相关案例.
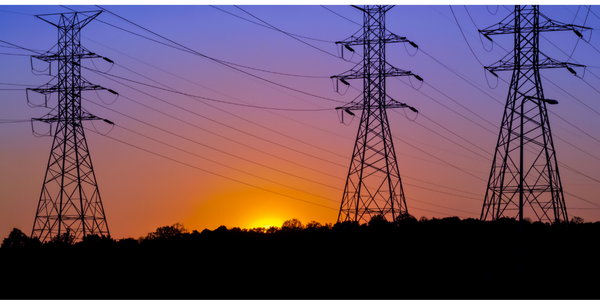
Case Study
Largest Production Deployment of AI and IoT Applications
To increase efficiency, develop new services, and spread a digital culture across the organization, Enel is executing an enterprise-wide digitalization strategy. Central to achieving the Fortune 100 company’s goals is the large-scale deployment of the C3 AI Suite and applications. Enel operates the world’s largest enterprise IoT system with 20 million smart meters across Italy and Spain.

Case Study
KeyBank's Digital Transformation with Confluent's Data in Motion
KeyBank, one of the nation's largest bank-based financial services companies, embarked on a national digital bank initiative following the acquisition of Laurel Road, a digital consumer lending business. The initiative aimed to build a digital bank focused on healthcare professionals looking to refinance student loans and buy homes. A significant challenge was reducing the time to market for new products by democratizing data and decoupling systems across the IT landscape. Like many large enterprises, KeyBank had a variety of vendor applications, custom applications, and other systems that were tightly coupled to one another. New projects often required developing specific point-to-point integrations for exchanging data, which did not address the needs of other downstream systems that could benefit from the same data.
Case Study
Bank BRI: Revolutionizing Financial Inclusion in Asia with Digital Banking
Bank Rakyat Indonesia (Bank BRI), one of the largest banks in Indonesia, was faced with the challenge of increasing financial inclusion among unbanked Indonesians. The bank had an ambitious target of having 84 percent of Indonesians participating in the banking system by 2022. However, the bank's legacy technologies were proving to be a hindrance in achieving this goal. Each of the bank's products had their own public APIs, which were difficult to manage, secure, and monetize. Additionally, the process of onboarding new partners using host-to-host and VPN technology was time-consuming, taking up to six months. The bank also faced the challenge of reaching a largely rural population, with an estimated $8.3 billion in currency being held outside the banking system.
Case Study
Neobank Transformation: Enhancing Compliance and Security
The client, a leading specialist digital challenger bank based in the UK, was faced with the challenge of redesigning and rebuilding their mobile banking application. The goal was to provide a more convenient way for their customers, primarily small businesses, entrepreneurs, and consumers, to interact with their platform. Additionally, they needed to implement Open Banking, a mandatory requirement from the UK financial institution. Prior to this, the client had outsourced the development of its mobile app to other vendors. However, they needed a strong team that would take over the development completely and implement new features to improve the functionality for both the client and its customers.
Case Study
Increasing Efficiency Through Automation and Modernization for Boohoo Group
Boohoo Group, a leading British online fashion retailer, faced significant challenges due to rapid growth and acquisition of other retailers. The company needed to modernize several internal systems used for warehouse management and tax calculation to maintain efficiency. The existing systems were causing data discrepancies and issues in product tracking. Additionally, a lot of data was stored in Excel files and had to be processed manually, which slowed down operations and increased expenses. The company aimed to automate these manual processes and modernize the existing solutions to boost their efficiency.
Case Study
Aerospike Achieves One Million Writes Per Second on Google Compute Engine with Just 50 Nodes
Aerospike, an open-source, flash-optimized, in-memory NoSQL database, was looking to push the boundaries of Google's speed on Google Compute Engine. The challenge was to meet high throughput, consistently low latency, and real-time processing, which are characteristic of future cloud applications. The team at Aerospike was inspired by Ivan Santa Maria Filho, Performance Engineering Lead at Google, who demonstrated 1 Million Writes Per Second with Cassandra on Google Compute Engine. The goal was to benchmark Aerospike's product performance on Google Compute Engine and see if it could scale with consistently low latency, require smaller clusters, and be simpler to operate.