Stanford Medicine Uses Snorkel to Revolutionize Medical Imaging Data Labeling
公司规模
1,000+
地区
- America
国家
- United States
产品
- Snorkel
技术栈
- Cross-modal Snorkel pipeline
实施规模
- Pilot projects
影响指标
- Cost Savings
- Digital Expertise
- Productivity Improvements
技术
- 分析与建模 - 机器学习
- 分析与建模 - 预测分析
适用行业
- 医疗保健和医院
- 生命科学
适用功能
- 产品研发
- 质量保证
用例
- 自动化疾病诊断
- 临床图像分析
- 远程病人监护
服务
- 数据科学服务
- 系统集成
关于客户
Stanford Medicine is a leading academic medical center that integrates research, medical education, and healthcare. Known for its cutting-edge research and innovative approaches to medical challenges, Stanford Medicine collaborates with various institutions to advance the field of medicine. The institution is committed to improving patient care through the development and application of new technologies. In this case, Stanford Medicine partnered with Snorkel to enhance their data labeling processes for medical imaging, aiming to improve the efficiency and accuracy of their machine learning models used in disease diagnosis and patient monitoring.
挑战
Labeling training data for triaging models in medical imaging is a time-consuming process, often requiring person-months to person-years of radiologist time. This manual labeling is not only labor-intensive but also prone to human error, which can affect the accuracy and reliability of the models. The challenge was to find a more efficient and accurate method to label large datasets of medical images, which are crucial for developing and training machine learning models for disease diagnosis and patient monitoring.
解决方案
Stanford Medicine deployed a cross-modal Snorkel pipeline to automate the labeling of medical imaging datasets. This innovative approach allowed them to replace the traditional manual labeling process, which took person-months to person-years, with a more efficient method that completed the task in just a few hours. The Snorkel pipeline was able to match or even exceed the performance of manually gathered labels, ensuring high accuracy and reliability. This solution not only saved significant time and resources but also improved the overall quality of the labeled data, which is essential for training effective machine learning models.
运营影响
数量效益
Case Study missing?
Start adding your own!
Register with your work email and create a new case study profile for your business.
相关案例.
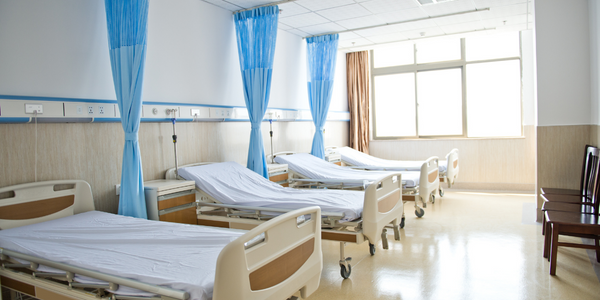
Case Study
Hospital Inventory Management
The hospital supply chain team is responsible for ensuring that the right medical supplies are readily available to clinicians when and where needed, and to do so in the most efficient manner possible. However, many of the systems and processes in use at the cancer center for supply chain management were not best suited to support these goals. Barcoding technology, a commonly used method for inventory management of medical supplies, is labor intensive, time consuming, does not provide real-time visibility into inventory levels and can be prone to error. Consequently, the lack of accurate and real-time visibility into inventory levels across multiple supply rooms in multiple hospital facilities creates additional inefficiency in the system causing over-ordering, hoarding, and wasted supplies. Other sources of waste and cost were also identified as candidates for improvement. Existing systems and processes did not provide adequate security for high-cost inventory within the hospital, which was another driver of cost. A lack of visibility into expiration dates for supplies resulted in supplies being wasted due to past expiry dates. Storage of supplies was also a key consideration given the location of the cancer center’s facilities in a dense urban setting, where space is always at a premium. In order to address the challenges outlined above, the hospital sought a solution that would provide real-time inventory information with high levels of accuracy, reduce the level of manual effort required and enable data driven decision making to ensure that the right supplies were readily available to clinicians in the right location at the right time.
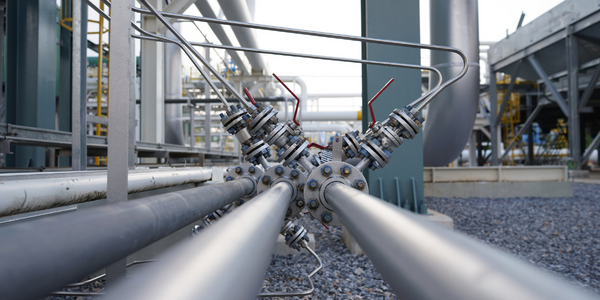
Case Study
Gas Pipeline Monitoring System for Hospitals
This system integrator focuses on providing centralized gas pipeline monitoring systems for hospitals. The service they provide makes it possible for hospitals to reduce both maintenance and labor costs. Since hospitals may not have an existing network suitable for this type of system, GPRS communication provides an easy and ready-to-use solution for remote, distributed monitoring systems System Requirements - GPRS communication - Seamless connection with SCADA software - Simple, front-end control capability - Expandable I/O channels - Combine AI, DI, and DO channels
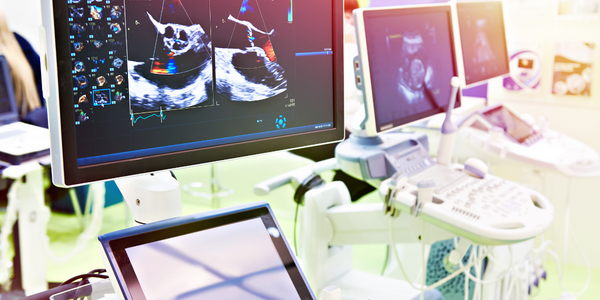
Case Study
Driving Digital Transformations for Vitro Diagnostic Medical Devices
Diagnostic devices play a vital role in helping to improve healthcare delivery. In fact, an estimated 60 percent of the world’s medical decisions are made with support from in vitrodiagnostics (IVD) solutions, such as those provided by Roche Diagnostics, an industry leader. As the demand for medical diagnostic services grows rapidly in hospitals and clinics across China, so does the market for IVD solutions. In addition, the typically high cost of these diagnostic devices means that comprehensive post-sales services are needed. Wanteed to improve three portions of thr IVD:1. Remotely monitor and manage IVD devices as fixed assets.2. Optimizing device availability with predictive maintenance.3. Recommending the best IVD solution for a customer’s needs.
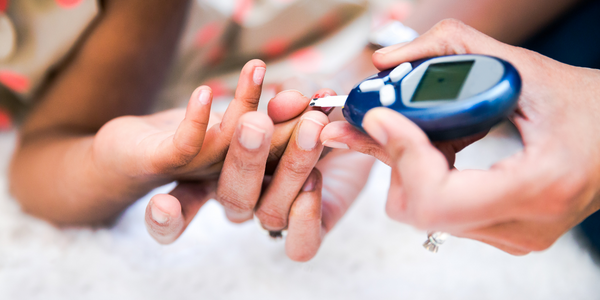
Case Study
HaemoCloud Global Blood Management System
1) Deliver a connected digital product system to protect and increase the differentiated value of Haemonetics blood and plasma solutions. 2) Improve patient outcomes by increasing the efficiency of blood supply flows. 3) Navigate and satisfy a complex web of global regulatory compliance requirements. 4) Reduce costly and labor-intensive maintenance procedures.
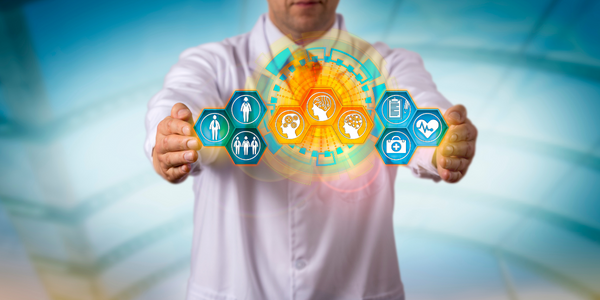
Case Study
Harnessing real-time data to give a holistic picture of patient health
Every day, vast quantities of data are collected about patients as they pass through health service organizations—from operational data such as treatment history and medications to physiological data captured by medical devices. The insights hidden within this treasure trove of data can be used to support more personalized treatments, more accurate diagnosis and more advanced preparative care. But since the information is generated faster than most organizations can consume it, unlocking the power of this big data can be a struggle. This type of predictive approach not only improves patient care—it also helps to reduce costs, because in the healthcare industry, prevention is almost always more cost-effective than treatment. However, collecting, analyzing and presenting these data-streams in a way that clinicians can easily understand can pose a significant technical challenge.