公司规模
SME
地区
- America
国家
- Canada
产品
- CovidWisdom app
- Pachyderm
技术栈
- Machine Learning
- AI architecture
- Data Science
- Docker containers
实施规模
- Enterprise-wide Deployment
影响指标
- Productivity Improvements
- Innovation Output
技术
- 分析与建模 - 机器学习
- 应用基础设施与中间件 - 数据交换与集成
适用行业
- 医疗保健和医院
- 城市与自治市
适用功能
- 商业运营
用例
- 预测性维护
- 公共交通管理
服务
- 数据科学服务
关于客户
Riskthinking.AI 是一家专门衡量气候变化财务风险的公司。他们与公司和政府合作,帮助他们在不确定的未来做出最佳决策。例如,他们可以帮助电力公司决定是否在过去引起森林火灾的同一地点重建变压器,或者将其置于不同的配置中,以减少未来再次引发火灾的可能性。他们还帮助公司确定他们能够以多快的速度扩建太阳能发电场以及应该将其建在何处。然而,他们很早就意识到,虽然他们有预测未来的专家,但他们缺乏构建 AI 架构的专业知识。
挑战
Riskthinking.AI 是一家专门衡量气候变化财务风险的公司,在接手 CovidWisdom 项目时,该公司正处于加强内部 AI 基础设施的早期阶段。该项目响应了加拿大政府的号召,旨在评估主要疫情政策对经济的影响。该项目的挑战在于预测实施社会层面应对措施(如封锁)的最佳方式,同时将对日常生活和经济的损害降至最低。然而,该团队意识到,他们有预测未来的专家,但没有构建 AI 架构的专家。他们让数据科学家使用笔记本电脑工作,通过 VPN 将数据拉取和推送到远程工作点,甚至构建自己的 Docker 容器。他们需要从临时转向 MLOps。
解决方案
Riskthinking.AI 决定使用 Pachyderm,该平台允许其科学家专注于模型的复杂性,而不是弄清楚哪个模型是在哪个版本的数据集上训练的复杂性。它为他们提供了处理数据和在机器学习循环中部署任何所需 ML 工具的基础。随着 Riskthinking.AI 的数据科学家对 pachctl 和命令行越来越熟悉,他们使用 Pachyderm 同时运行多个模型,并通过易于理解的图像可视化回测结果。当天表现最佳的模型会自动推送到应用程序中。可视化不仅适用于数据科学团队。他们还可以与非技术或技术水平较低的利益相关者分享他们的进展。
运营影响
数量效益
Case Study missing?
Start adding your own!
Register with your work email and create a new case study profile for your business.
相关案例.
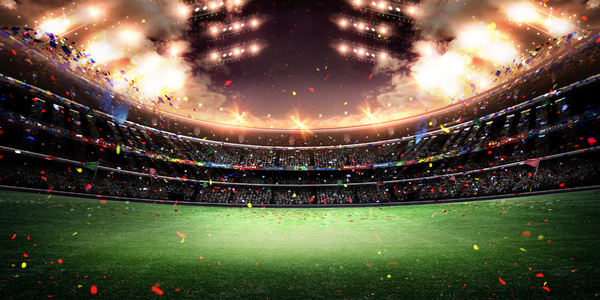
Case Study
Turning A Stadium Into A Smart Building
Honeywell created what it called the “intelligent system” for the National Stadium in Beijing, China, turning the venue for the opening and closing events at the 2008 Summer Olympics into a “smart building.” Designed by highly controversial artist Ai Weiwei, the “Bird’s Nest” remains one of the most impressive feats of stadium architecture in the world. The 250,000 square meter structure housed more than 100,000 athletes and spectators at a time. To accommodate such capacity, China turned to Honeywell’s EBI Integrated Building Management System to create an integrated “intelligent system” for improved building security, safety and energy efficiency.
.png)
Case Study
Smart Street Light Network (Copenhagen)
Key stakeholders are taking a comprehensive approach to rethinking smart city innovation. City leaders have collaborated through partnerships involving government, research institutions and solution providers. The Copenhagen Solutions Lab is one of the leading organizations at the forefront of this movement. By bringing together manufacturers with municipal buyers, the Copenhagen Solutions Lab has catalyzed the development and deployment of next-generation smart city innovations. Copenhagen is leveraging this unique approach to accelerate the implementation of smart city solutions. One of the primary focus areas is LED street lighting.
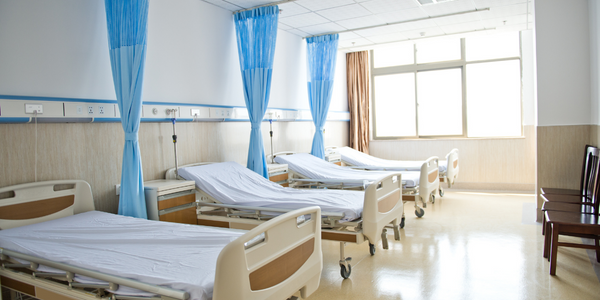
Case Study
Hospital Inventory Management
The hospital supply chain team is responsible for ensuring that the right medical supplies are readily available to clinicians when and where needed, and to do so in the most efficient manner possible. However, many of the systems and processes in use at the cancer center for supply chain management were not best suited to support these goals. Barcoding technology, a commonly used method for inventory management of medical supplies, is labor intensive, time consuming, does not provide real-time visibility into inventory levels and can be prone to error. Consequently, the lack of accurate and real-time visibility into inventory levels across multiple supply rooms in multiple hospital facilities creates additional inefficiency in the system causing over-ordering, hoarding, and wasted supplies. Other sources of waste and cost were also identified as candidates for improvement. Existing systems and processes did not provide adequate security for high-cost inventory within the hospital, which was another driver of cost. A lack of visibility into expiration dates for supplies resulted in supplies being wasted due to past expiry dates. Storage of supplies was also a key consideration given the location of the cancer center’s facilities in a dense urban setting, where space is always at a premium. In order to address the challenges outlined above, the hospital sought a solution that would provide real-time inventory information with high levels of accuracy, reduce the level of manual effort required and enable data driven decision making to ensure that the right supplies were readily available to clinicians in the right location at the right time.
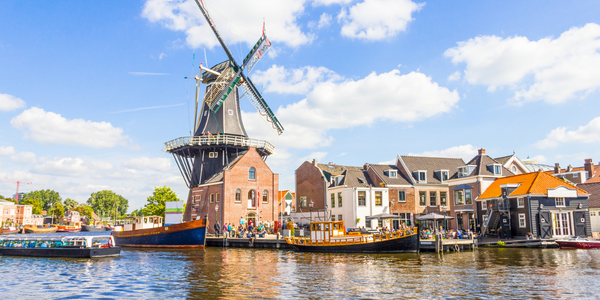
Case Study
Buoy Status Monitoring with LoRa
The Netherlands are well-known for their inland waterways, canals, sluices and of course port activities. The Dutch Ministry of Infrastructure indicates that there are thousands of buoys and fixed items in and near water environments that would profit from IoT monitoring. One of the problems with buoys for example, is that they get hit by ships and the anchor cable breaks. Without connectivity, it takes quite some time to find out that something has happened with that buoy. Not to mention the costs of renting a boat to go to the buoy to fix it. Another important issue, is that there is no real-time monitoring of the buoys at this moment. Only by physically visiting the object on the water, one gains insight in its status.
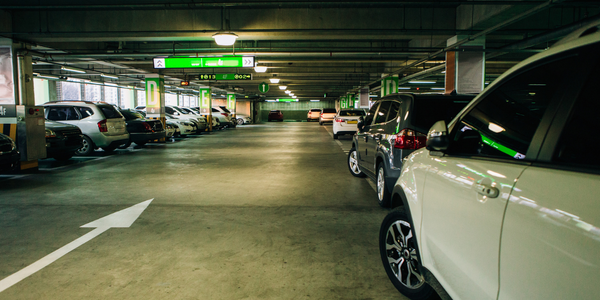
Case Study
China Mobile Smart Parking
Smart Parking, powered by NB-IoT technology, is making it easier for drivers to find free parking spots. Cities can better manage their parking assets and maximize the revenue available to them as a result. Drivers searching for parking create congestion and pollution by circling and hunting for available parking. Smart Parking services are able to significantly ease these problems by guiding a driver directly to a parking space.