公司规模
1,000+
地区
- America
国家
- United States
产品
- Advanced Alert Monitoring System
技术栈
- Machine Learning
- Data Analytics
- H2O
实施规模
- Enterprise-wide Deployment
影响指标
- Customer Satisfaction
- Productivity Improvements
技术
- 分析与建模 - 机器学习
- 分析与建模 - 预测分析
适用行业
- 医疗保健和医院
适用功能
- 质量保证
用例
- 预测性维护
- 远程病人监护
服务
- 数据科学服务
关于客户
Kaiser Permanente is an integrated healthcare system, where patients receive a variety of services and treatments under one roof. With 10 million members, Kaiser Permanente has vast amounts of data, and is using innovative approaches to make this data work for the benefit of its patients and clinicians. Kaiser has always been on the forefront of using technology – as early as 1970s, the company began keeping electronic medical records. Today, Kaiser members have a variety of methods available to them to interact with their doctors and nurses – using the web, e-mail and mobile apps to get the answers they need faster. All this data is captured and can be used to solve specific problems.
挑战
Kaiser Permanente, an integrated healthcare system, was facing a challenge with patients who undergo an unplanned transfer to the Intensive Care Unit (ICU). These patients experienced significantly worse outcomes than those directly admitted to the ICU. They represented about a fourth of all Kaiser ICU admissions, a fifth of all deaths in the hospital, and about an eighth of all of the hospital days. The patients who experienced an unplanned transfer to the ICU experienced two to five times the mortality of patients who are directly admitted to the ICU, and they would stay in the hospital an average of 8 to 12 days more than patients who are directly admitted to the ICU. The challenge was to identify these patients ahead of time who are likely to crash and be rushed to the ICU, and intervene before the deterioration.
解决方案
Kaiser decided to use available data about a patient and build a mechanism that could identify when deterioration might occur. They developed the Advanced Alert Monitoring (AAM) System, which helps identify patients who are likely to crash, and they recognize them 12 hours before they experience the deterioration. The AAM has four components: risk analysis and the creation of the score, monitoring and warning, dissemination and communication of the scope, and finally – the intervention. They used bed history data, chemistry, vital signs, comorbidities, and demographic information to estimate either the probability of a late transferring to the ICU that is a sudden crash or mortality in the hospital. Once the data is collected and cleaned, the team worked on feature engineering and then applied a variety of training and testing datasets. The team explored each parameter and its linkage, got the clinicians involved to look at every case, and then performed a characteristics review and threshold analysis to make sure that a threshold made sense.
运营影响
数量效益
Case Study missing?
Start adding your own!
Register with your work email and create a new case study profile for your business.
相关案例.
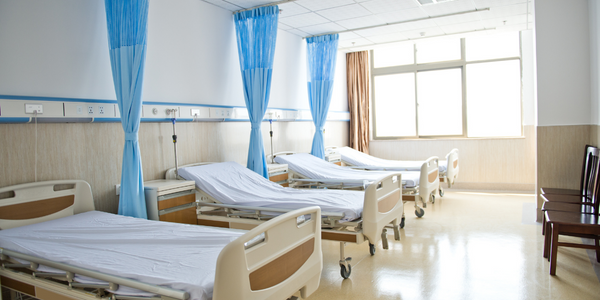
Case Study
Hospital Inventory Management
The hospital supply chain team is responsible for ensuring that the right medical supplies are readily available to clinicians when and where needed, and to do so in the most efficient manner possible. However, many of the systems and processes in use at the cancer center for supply chain management were not best suited to support these goals. Barcoding technology, a commonly used method for inventory management of medical supplies, is labor intensive, time consuming, does not provide real-time visibility into inventory levels and can be prone to error. Consequently, the lack of accurate and real-time visibility into inventory levels across multiple supply rooms in multiple hospital facilities creates additional inefficiency in the system causing over-ordering, hoarding, and wasted supplies. Other sources of waste and cost were also identified as candidates for improvement. Existing systems and processes did not provide adequate security for high-cost inventory within the hospital, which was another driver of cost. A lack of visibility into expiration dates for supplies resulted in supplies being wasted due to past expiry dates. Storage of supplies was also a key consideration given the location of the cancer center’s facilities in a dense urban setting, where space is always at a premium. In order to address the challenges outlined above, the hospital sought a solution that would provide real-time inventory information with high levels of accuracy, reduce the level of manual effort required and enable data driven decision making to ensure that the right supplies were readily available to clinicians in the right location at the right time.
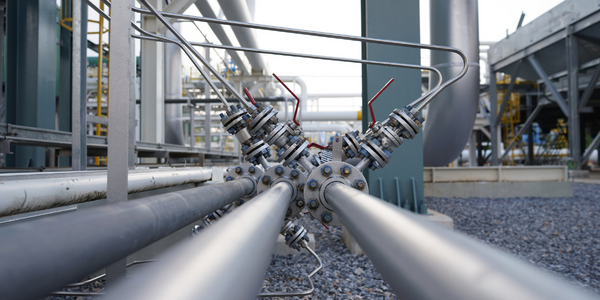
Case Study
Gas Pipeline Monitoring System for Hospitals
This system integrator focuses on providing centralized gas pipeline monitoring systems for hospitals. The service they provide makes it possible for hospitals to reduce both maintenance and labor costs. Since hospitals may not have an existing network suitable for this type of system, GPRS communication provides an easy and ready-to-use solution for remote, distributed monitoring systems System Requirements - GPRS communication - Seamless connection with SCADA software - Simple, front-end control capability - Expandable I/O channels - Combine AI, DI, and DO channels
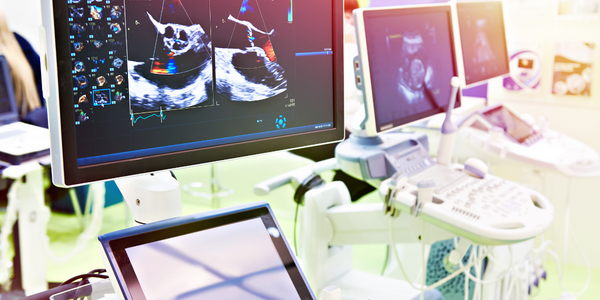
Case Study
Driving Digital Transformations for Vitro Diagnostic Medical Devices
Diagnostic devices play a vital role in helping to improve healthcare delivery. In fact, an estimated 60 percent of the world’s medical decisions are made with support from in vitrodiagnostics (IVD) solutions, such as those provided by Roche Diagnostics, an industry leader. As the demand for medical diagnostic services grows rapidly in hospitals and clinics across China, so does the market for IVD solutions. In addition, the typically high cost of these diagnostic devices means that comprehensive post-sales services are needed. Wanteed to improve three portions of thr IVD:1. Remotely monitor and manage IVD devices as fixed assets.2. Optimizing device availability with predictive maintenance.3. Recommending the best IVD solution for a customer’s needs.
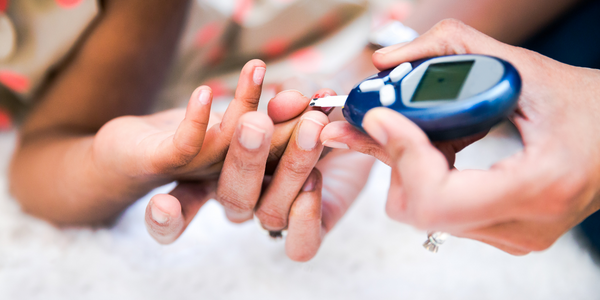
Case Study
HaemoCloud Global Blood Management System
1) Deliver a connected digital product system to protect and increase the differentiated value of Haemonetics blood and plasma solutions. 2) Improve patient outcomes by increasing the efficiency of blood supply flows. 3) Navigate and satisfy a complex web of global regulatory compliance requirements. 4) Reduce costly and labor-intensive maintenance procedures.
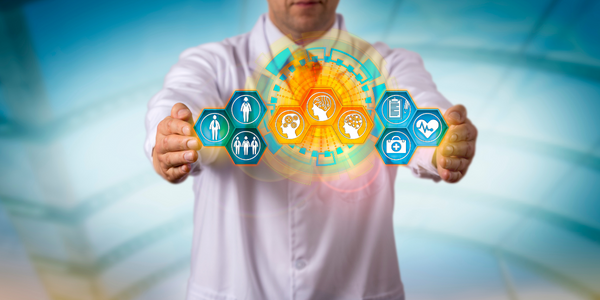
Case Study
Harnessing real-time data to give a holistic picture of patient health
Every day, vast quantities of data are collected about patients as they pass through health service organizations—from operational data such as treatment history and medications to physiological data captured by medical devices. The insights hidden within this treasure trove of data can be used to support more personalized treatments, more accurate diagnosis and more advanced preparative care. But since the information is generated faster than most organizations can consume it, unlocking the power of this big data can be a struggle. This type of predictive approach not only improves patient care—it also helps to reduce costs, because in the healthcare industry, prevention is almost always more cost-effective than treatment. However, collecting, analyzing and presenting these data-streams in a way that clinicians can easily understand can pose a significant technical challenge.