公司规模
200-1,000
地区
- America
国家
- Canada
产品
- Sift
技术栈
- Machine Learning
- API
实施规模
- Enterprise-wide Deployment
影响指标
- Cost Savings
- Productivity Improvements
技术
- 分析与建模 - 机器学习
适用行业
- 零售
适用功能
- 销售与市场营销
用例
- 欺诈识别
服务
- 数据科学服务
关于客户
Dbrand is a leader in the custom skin market, offering shoppers the opportunity to personalize their countless gadgets with unique, customizable, and precision-fitted vinyl wraps. All of their products are developed and manufactured in Toronto. Dbrand's distinctive and industry-changing virtual skin building interface puts the creative power in the hands of consumers. As their business grew, they faced an increasing number of fraudsters on their site, leading to costly chargebacks and hours of manual review. They needed a smarter, more scalable solution to mitigate the impact of fraud on their bottom line and brand.
挑战
As dbrand's business grew, so did the number of fraudsters creeping onto their site. The majority of the fraud they experienced was from bad users purchasing goods using stolen credit cards. The resulting chargebacks were costly, not only due to the high-quality product that was lost, the sale that was refunded, or the bank-levied chargeback fees, but also the hours of manual review and headaches that the fraud caused. Even as their chargeback rate reached a high of 2.18% in a single month and 4 customer service employees became dedicated fraud management experts, fraudsters continued to slip past their defenses. To mitigate the impact of fraud on their bottom line and brand, dbrand sought a smarter and more scalable solution.
解决方案
After researching fraud management solutions, dbrand CEO Adam Ijaz was disappointed to find that many required ongoing manual review and hand-holding. In search of a vendor that could reduce their workload by growing efficiency, Adam discovered Sift, drawn by the product’s machine learning and automation features. Full integration took a week, and was extremely simple with Sift’s easy API and extensive documentation. With just one month of training, dbrand’s custom machine learning algorithms were catching fraud unique to the business, identifying returning and new fraudsters alike.
运营影响
数量效益
Case Study missing?
Start adding your own!
Register with your work email and create a new case study profile for your business.
相关案例.
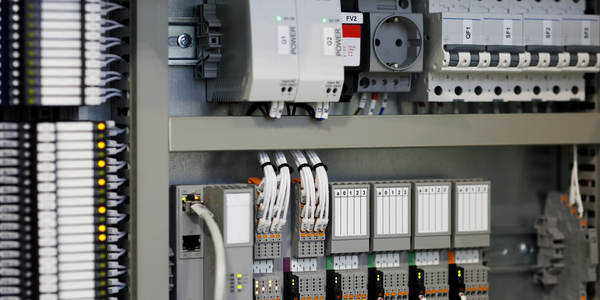
Case Study
Improving Production Line Efficiency with Ethernet Micro RTU Controller
Moxa was asked to provide a connectivity solution for one of the world's leading cosmetics companies. This multinational corporation, with retail presence in 130 countries, 23 global braches, and over 66,000 employees, sought to improve the efficiency of their production process by migrating from manual monitoring to an automatic productivity monitoring system. The production line was being monitored by ABB Real-TPI, a factory information system that offers data collection and analysis to improve plant efficiency. Due to software limitations, the customer needed an OPC server and a corresponding I/O solution to collect data from additional sensor devices for the Real-TPI system. The goal is to enable the factory information system to more thoroughly collect data from every corner of the production line. This will improve its ability to measure Overall Equipment Effectiveness (OEE) and translate into increased production efficiencies. System Requirements • Instant status updates while still consuming minimal bandwidth to relieve strain on limited factory networks • Interoperable with ABB Real-TPI • Small form factor appropriate for deployment where space is scarce • Remote software management and configuration to simplify operations
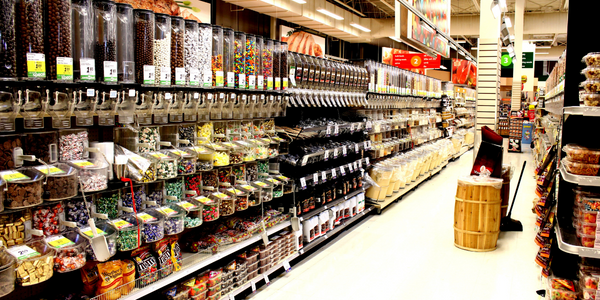
Case Study
How Sirqul’s IoT Platform is Crafting Carrefour’s New In-Store Experiences
Carrefour Taiwan’s goal is to be completely digital by end of 2018. Out-dated manual methods for analysis and assumptions limited Carrefour’s ability to change the customer experience and were void of real-time decision-making capabilities. Rather than relying solely on sales data, assumptions, and disparate systems, Carrefour Taiwan’s CEO led an initiative to find a connected IoT solution that could give the team the ability to make real-time changes and more informed decisions. Prior to implementing, Carrefour struggled to address their conversion rates and did not have the proper insights into the customer decision-making process nor how to make an immediate impact without losing customer confidence.
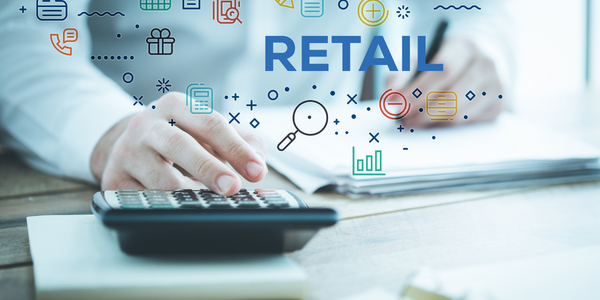
Case Study
Digital Retail Security Solutions
Sennco wanted to help its retail customers increase sales and profits by developing an innovative alarm system as opposed to conventional connected alarms that are permanently tethered to display products. These traditional security systems were cumbersome and intrusive to the customer shopping experience. Additionally, they provided no useful data or analytics.
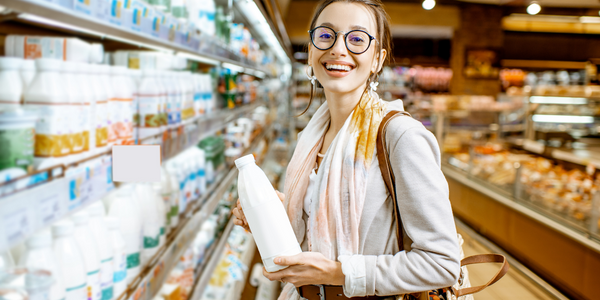
Case Study
Ensures Cold Milk in Your Supermarket
As of 2014, AK-Centralen has over 1,500 Danish supermarkets equipped, and utilizes 16 operators, and is open 24 hours a day, 365 days a year. AK-Centralen needed the ability to monitor the cooling alarms from around the country, 24 hours a day, 365 days a year. Each and every time the door to a milk cooler or a freezer does not close properly, an alarm goes off on a computer screen in a control building in southwestern Odense. This type of alarm will go off approximately 140,000 times per year, equating to roughly 400 alarms in a 24-hour period. Should an alarm go off, then there is only a limited amount of time to act before dairy products or frozen pizza must be disposed of, and this type of waste can quickly start to cost a supermarket a great deal of money.
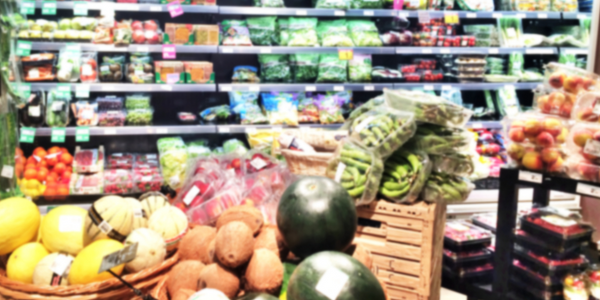
Case Study
Supermarket Energy Savings
The client had previously deployed a one-meter-per-store monitoring program. Given the manner in which energy consumption changes with external temperature, hour of the day, day of week and month of year, a single meter solution lacked the ability to detect the difference between a true problem and a changing store environment. Most importantly, a single meter solution could never identify root cause of energy consumption changes. This approach never reduced the number of truck-rolls or man-hours required to find and resolve issues.