Georgetown University's CSET Accelerates AI Development with Snorkel Flow
公司规模
1,000+
地区
- America
国家
- United States
产品
- Snorkel Flow
技术栈
- NLP
- Active Learning
实施规模
- Enterprise-wide Deployment
影响指标
- Customer Satisfaction
- Digital Expertise
- Productivity Improvements
技术
- 分析与建模 - 机器学习
- 分析与建模 - 自然语言处理 (NLP)
适用行业
- 教育
- Software
适用功能
- 产品研发
- 质量保证
用例
- 自动化疾病诊断
- 质量预测分析
服务
- 数据科学服务
- 软件设计与工程服务
关于客户
Georgetown University's Center for Security and Emerging Technology (CSET) is a leading research organization focused on studying the security implications of emerging technologies. The center aims to provide policymakers with data-driven analysis and recommendations to navigate the complex landscape of technological advancements. CSET's data science team is dedicated to developing advanced AI models to classify and analyze scientific articles, particularly in the field of virology. The team faced significant challenges in managing the manual labeling workflows required for their NLP applications, which led them to seek more efficient and scalable solutions.
挑战
CSET's data science team wanted to build NLP applications that classify scientific articles such as technical papers on virology. The team realized that manual labeling workflows would be impractical for the task.
解决方案
With the help of integrated analysis tools within Snorkel Flow, the team was able to pinpoint data slices for domain expert spot-checks and troubleshooting to improve accuracy. This approach powered an active learning workflow, significantly enhancing the efficiency of their labeling process. Snorkel Flow's advanced features, such as autosuggest and cluster labeling functions (LFs), enabled the team to create 107,000 programmatic labels. This not only reduced the labeling time by 50% but also improved productivity and accuracy. Within days, the team achieved 85% accuracy on a classification model, demonstrating the effectiveness of the solution.
运营影响
数量效益
Case Study missing?
Start adding your own!
Register with your work email and create a new case study profile for your business.
相关案例.
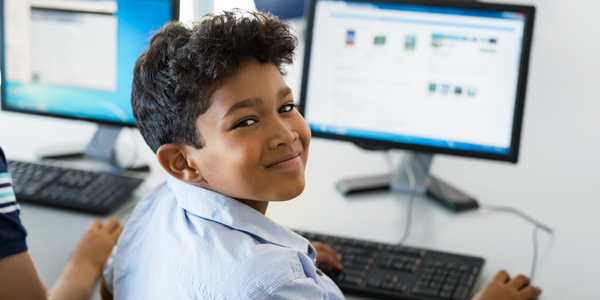
Case Study
IoT platform Enables Safety Solutions for U.S. School Districts
Designed to alert drivers when schoolchildren are present, especially in low-visibility conditions, school-zone flasher signals are typically updated manually at each school. The switching is based on the school calendar and manually changed when an unexpected early dismissal occurs, as in the case of a weather-event altering the normal schedule. The process to reprogram the flashers requires a significant effort by school district personnel to implement due to the large number of warning flashers installed across an entire school district.
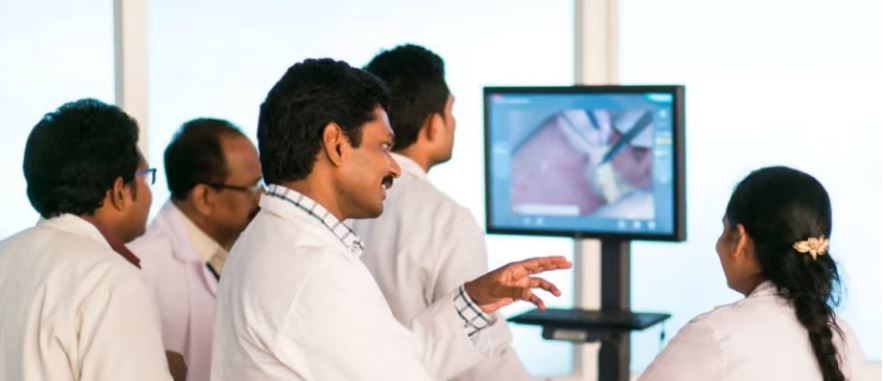
Case Study
Revolutionizing Medical Training in India: GSL Smart Lab and the LAP Mentor
The GSL SMART Lab, a collective effort of the GSL College of Medicine and the GSL College of Nursing and Health Science, was facing a challenge in providing superior training to healthcare professionals. As clinical medicine was becoming more focused on patient safety and quality of care, the need for medical simulation to bridge the educational gap between the classroom and the clinical environment was becoming increasingly apparent. Dr. Sandeep Ganni, the director of the GSL SMART Lab, envisioned a world-class surgical and medical training center where physicians and healthcare professionals could learn skills through simulation training. He was looking for different simulators for different specialties to provide both basic and advanced simulation training. For laparoscopic surgery, he was interested in a high fidelity simulator that could provide basic surgical and suturing skills training for international accreditation as well as specific hands-on training in complex laparoscopic procedures for practicing physicians in India.
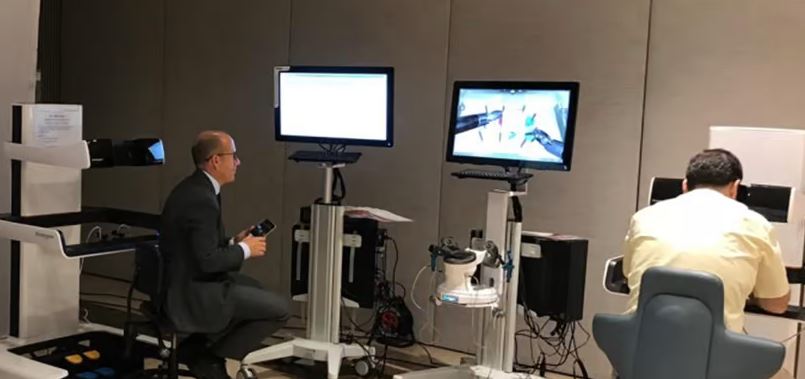
Case Study
Implementing Robotic Surgery Training Simulator for Enhanced Surgical Proficiency
Fundacio Puigvert, a leading European medical center specializing in Urology, Nephrology, and Andrology, faced a significant challenge in training its surgical residents. The institution recognized the need for a more standardized and comprehensive training curriculum, particularly in the area of robotic surgery. The challenge was underscored by two independent studies showing that less than 5% of residents in Italian and German residency programs could perform major or complex procedures by the end of their residency. The institution sought to establish a virtual reality simulation lab that would include endourological, laparoscopic, and robotic platforms. However, they needed a simulator that could replicate both the hardware and software of the robotic Da Vinci console used in the operating room, without being connected to the actual physical console. They also required a system that could provide both basic and advanced simulation training, and a metrics system to assess the proficiency of the trainees before they performed surgical procedures in the operating theater.
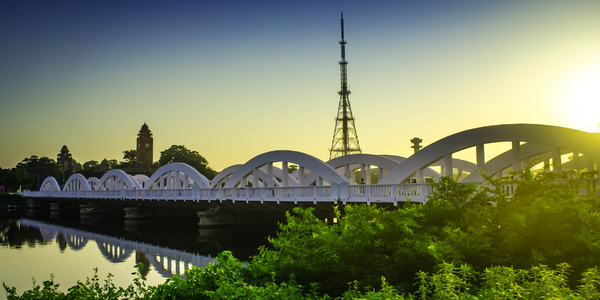
Case Study
Edinburgh Napier University streamlines long-distance learning with Cisco WebEX
• Geographically dispersed campus made in-person meetings costly and inconvenient.• Distance-learning programs in Malaysia, India, and China required dependable, user-friendly online tools to maximize interaction in collaborative workspaces.• Virtual learning environment required a separate sign-in process, resulting in a significant administrative burden for IT staff and limited adoption of collaboration technology.
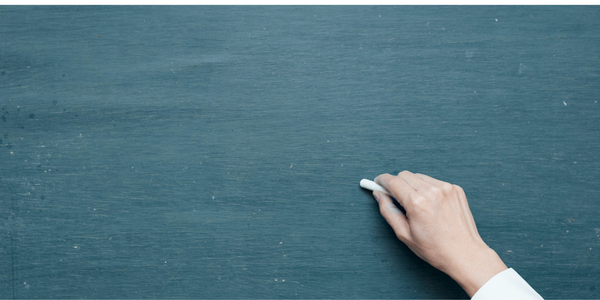
Case Study
8x increased productivity with VKS
Before VKS, a teacher would spend a lot of time showing a group of 22 students how to build a set of stairs within a semester of 120 hours. Along with not leaving the teacher much time to provide one-on-one support for each student to properly learn carpentry, it also left a considerable amount of room for error. Key information would be misinterpreted or lost as the class was taught in the typical show-and-tell way.
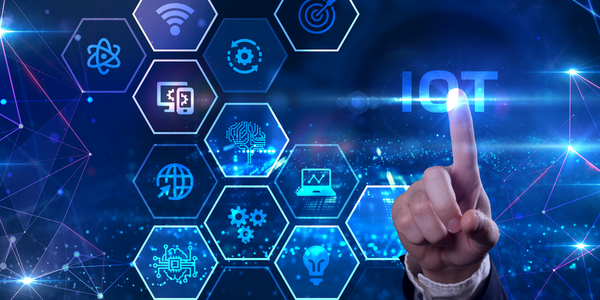
Case Study
Scalable IoT Empowering GreenFlex's Sustainable Growth
GreenFlex, a company that supports sustainable development, decarbonization, and energy efficiency, faced several challenges in its quest to expand its business. The company needed to deploy a robust and sustainable IoT technology to support its growth. It was crucial for them to monitor and control devices at customer sites in a safe and reliable manner. They also needed to integrate devices across a range of communication protocols and gather and act on data to meet efficiency targets. GreenFlex had previously built IoT capabilities into its digital platform, GreenFlexIQ, to monitor and manage customer sites remotely. However, they soon realized that they needed a new platform to support their ambitions. They needed a platform that could scale to connect more devices for production management and make it easier for the operations team to manage devices in the field.