Developed model to identify utility customers at risk of seasonal defection from Time-of-Use (TOU) price plan
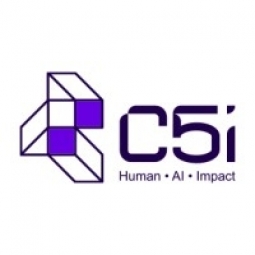
公司规模
1,000+
地区
- America
国家
- United States
产品
- Predictive Analytics Model
技术栈
- C5.0
- CHAID
- QUEST
- Logistic Regression
实施规模
- Enterprise-wide Deployment
影响指标
- Customer Satisfaction
- Productivity Improvements
技术
- 分析与建模 - 预测分析
适用行业
- 公用事业
适用功能
- 商业运营
用例
- 补货预测
服务
- 数据科学服务
关于客户
The customer is a large public utility company based in the United States. They provide essential utility services to a vast number of residential customers. The company offers various price plans to its customers, including a Time-of-Use (TOU) price plan. However, they were facing a challenge in identifying which customers on the TOU price plan were likely to switch to an alternate price plan. The company needed a solution that could accurately predict this switching behavior and help them retain their customers.
挑战
The client, a large US public utility, was facing a challenge in identifying which customers on a Time-of-Use (TOU) price plan would switch to an alternate price plan. They needed to understand the factors that predict this switching behavior and create propensity scores for each of the current TOU price plan customers to identify their individual likelihood of switching to a different price plan. The goal was to correctly predict a likely switcher approximately 70% of the time.
解决方案
Blueocean Market Intelligence developed a predictive analytics model using the client’s data repository of residential customer information and the Meter Data Management System over a two-year period. Historical and customer demographic information was used to predict program participation and identify differences between program switchers and non-switchers. Simultaneous models were run using C5.0, CHAID, QUEST, and logistic regression. The final commissioned model had a high level of predictive accuracy of 70% for who was most likely to switch.
运营影响
数量效益
Case Study missing?
Start adding your own!
Register with your work email and create a new case study profile for your business.
相关案例.

Case Study
IoT Solutions for Smart City | Internet of Things Case Study
There were several challenges faced: It is challenging to build an appliance that can withstand a wide range of voltage fluctuations from as low at 90v to as high as 320v. Since the device would be installed in remote locations, its resilience was of paramount importance. The device would have to deal with poor network coverage and have the ability to store and re-transmit data if networks were not available, which is often the case in rural India. The device could store up to 30 days of data.
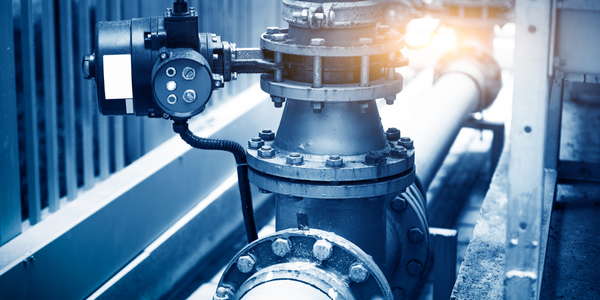
Case Study
Automation of the Oguz-Gabala-Baku water pipeline, Azerbaijan
The Oguz-Gabala-Baku water pipeline project dates back to plans from the 1970’s. Baku’s growth was historically driven by the booming oil industry and required the import of drinking water from outside of the city. Before the construction of the pipeline, some 60 percent of the city’s households received water for only a few hours daily. After completion of the project, 75 percent of the two million Baku residents are now served around the clock with potable water, based on World Health Organization (WHO) standards. The 262-kilometer pipeline requires no pumping station, but uses the altitude differences between the Caucasian mountains and the capital to supply 432,000 m³/d to the Ceyranbatan water reservoir. To the people of Baku, the pipeline is “the most important project not only in 2010, but of the last 20 years.”
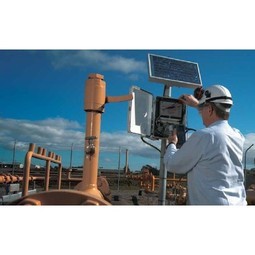
Case Study
GPRS Mobile Network for Smart Metering
Around the world, the electricity supply industry is turning to ‘smart’ meters to lower costs, reduce emissions and improve the management of customer supplies. Smart meters collect detailed consumption information and using this feedback consumers can better understand their energy usage which in turn enables them to modify their consumption to save money and help to cut carbon emissions. A smart meter can be defined in many ways, but generally includes an element of two-way communication between the household meter and the utility provider to efficiently collect detailed energy usage data. Some implementations include consumer feedback beyond the energy bill to include online web data, SMS text messages or an information display in consumers’ premises. Providing a cost-effective, reliable communications mechanism is one of the most challenging aspects of a smart meter implementation. In New Zealand, the utilities have embraced smart metering and designed cost effective ways for it to be implemented. The New Zealand government has encouraged such a move to smart metering by ensuring the energy legislation is consistent with the delivery of benefits to the consumer while allowing innovation in this area. On the ground, AMS is a leader in the deployment of smart metering and associated services. Several of New Zealand’s energy retailers were looking for smart metering services for their residential and small business customers which will eventually account for over 500,000 meters when the multi-year national deployment program is concluded. To respond to these requirements, AMS needed to put together a solution that included data communications between each meter and the central data collection point and the solution proposed by Vodafone satisfied that requirement.
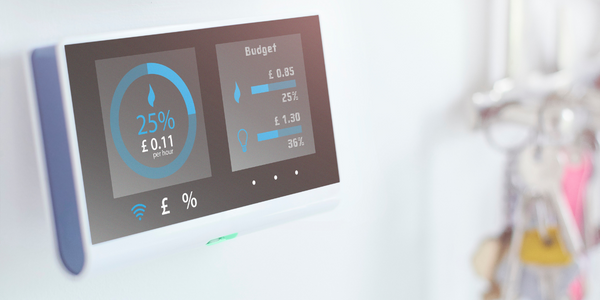
Case Study
NB-IoT connected smart meters to improve gas metering in Shenzhen
Shenzhen Gas has a large fleet of existing gas meters, which are installed in a variety of hard to reach locations, such as indoors and underground, meaning that existing communications networks have struggled to maintain connectivity with all meters. The meter success rate is low, data transmissions are so far unstable and power consumption is too high. Against this background, Shenzhen Gas, China Telecom, Huawei, and Goldcard have jointly trialed NB-IoT gas meters to try and solve some of the challenges that the industry faces with today’s smart gas meters.
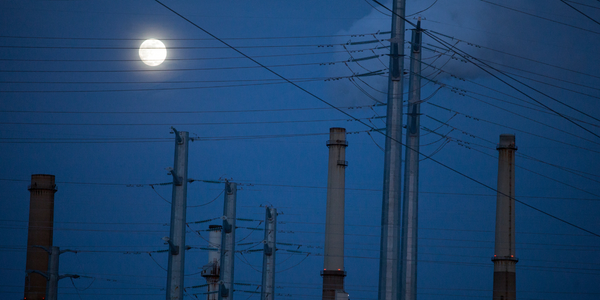
Case Study
OneWireless Enabled Performance Guarantee Test
Tata Power's power generation equipment OEMs (M/s BHEL) is required to provide all of the instrumentation and measurement devices for conducting performance guarantee and performance evaluation tests. M/s BHEL faced a number of specific challenges in conducting PG tests: employing high-accuracy digital communications for instrumentation, shortening setup and dismantling time, reducing hardware required, making portable instrument setup, avoiding temporary cabling work and the material waste costs
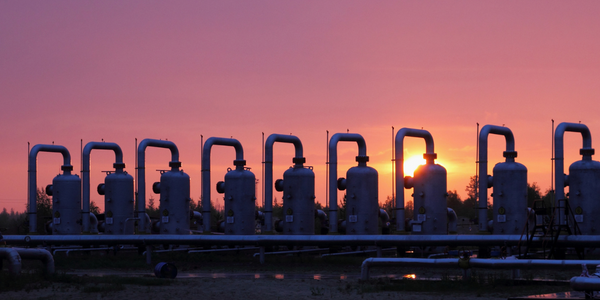
Case Study
British Gas Modernizes its Operations with Innovative Smart Metering Deployment
The UK government has mandated that smart meters are rolled out as standard across Great Britain by end of 2020, and this roll-out is estimated to create £14 billion in net benefits to the UK in consumer energy savings and lower energy generation demand, according to the Oxford Economics report, “The Value of Smart Metering to Great Britain.” While smart-metering systems have been deployed in many countries, the roll-out in Great Britain is unique because it is led by energy retailers, who have responsibility for the Electricity and Gas meters. The decision to have a retailer-led roll out was made by DECC (Department of Energy and Climate Change) to improve customer experience and drive consumer benefits. It has also led to some unique system-level requirements to support the unique local regulatory model.