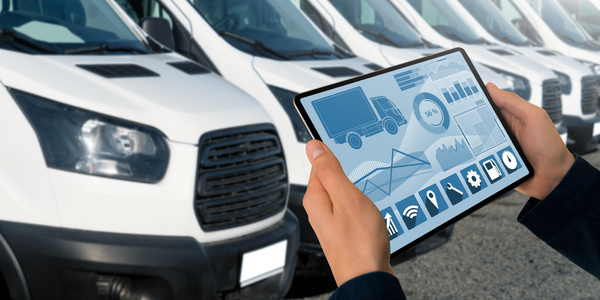
Technology Category
- Analytics & Modeling - Big Data Analytics
- Analytics & Modeling - Predictive Analytics
- Functional Applications - Fleet Management Systems (FMS)
- Infrastructure as a Service (IaaS)
Applicable Industries
- Transportation
Applicable Functions
- Logistics & Transportation
Use Cases
- Fleet Management
About The Customer
A global corporation in the transportation and logistics industry
The Challenge
Organizations frequently implement a maintenance strategy for their fleets of vehicles using a combination of time and usage based maintenance schedules. While effective as a whole, time and usage based schedules do not take into account driving patterns, environmental factors, and sensors currently deployed within the vehicle measuring crank voltage, ignition voltage, and acceleration, all of which have a significant influence on the overall health of the vehicle.
In a typical fleet, a large percentage of road calls are related to electrical failure, with battery failure being a common cause. Battery failures result in unmet service agreement levels and costly re-adjustment of scheduled to provide replacement vehicles. To reduce the impact of unplanned maintenance, the transportation logistics company was interested in a trial of C3 Vehicle Fleet Analytics.
The Solution
A global corporation in the transportation and logistics industry completed a trial of C3 Vehicle Fleet Analytics in less than one week, demonstrating the ability to rapidly develop big data predictive analytic applications on the C3 IoT Platform.
The trial was scoped to analyze 10,000 vehicles with historical maintenance records and 3 years of sensor data. C3 IoT first defined the data model to store vehicle and vehicle sensor data. Using the metadata based C3 Type System, C3 IoT rapidly defined the data and canonical object models and the transformations required to convert source objects to the C3 data model. This then enabled the C3 IoT team to rapidly ingest the sensor data for all 10,000 vehicles (approximately 1 TB) in under a day. Additionally, C3 IoT defined 26 time series analytics based on vehicle sensor data. These analytic were then fed into a machine learning classifier to predict battery failure.
With less than a week of work, the C3 IoT team:
• Developed a data and canonical objects model for vehicle operations
• Loaded a terabyte of vehicle and vehicle sensor data
• Developed 25 time series analytics
• Defined and executed a machine learning classifier across the entire data set to predict which vehicles would experience a battery failure
The trial was scoped to analyze 10,000 vehicles with historical maintenance records and 3 years of sensor data. C3 IoT first defined the data model to store vehicle and vehicle sensor data. Using the metadata based C3 Type System, C3 IoT rapidly defined the data and canonical object models and the transformations required to convert source objects to the C3 data model. This then enabled the C3 IoT team to rapidly ingest the sensor data for all 10,000 vehicles (approximately 1 TB) in under a day. Additionally, C3 IoT defined 26 time series analytics based on vehicle sensor data. These analytic were then fed into a machine learning classifier to predict battery failure.
With less than a week of work, the C3 IoT team:
• Developed a data and canonical objects model for vehicle operations
• Loaded a terabyte of vehicle and vehicle sensor data
• Developed 25 time series analytics
• Defined and executed a machine learning classifier across the entire data set to predict which vehicles would experience a battery failure
Quantitative Benefit
Case Study missing?
Start adding your own!
Register with your work email and create a new case study profile for your business.
Related Case Studies.
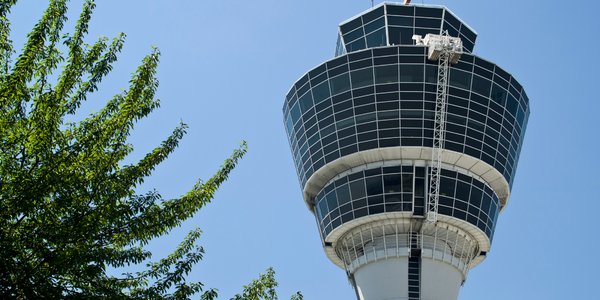
Case Study
Airport SCADA Systems Improve Service Levels
Modern airports are one of the busiest environments on Earth and rely on process automation equipment to ensure service operators achieve their KPIs. Increasingly airport SCADA systems are being used to control all aspects of the operation and associated facilities. This is because unplanned system downtime can cost dearly, both in terms of reduced revenues and the associated loss of customer satisfaction due to inevitable travel inconvenience and disruption.
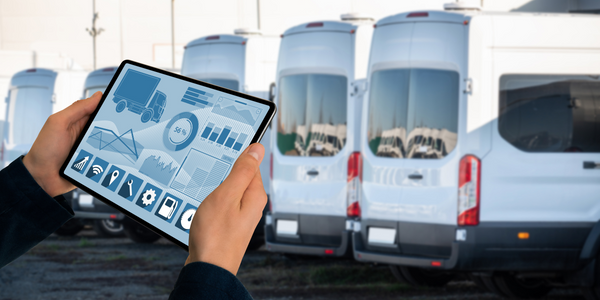
Case Study
IoT-based Fleet Intelligence Innovation
Speed to market is precious for DRVR, a rapidly growing start-up company. With a business model dependent on reliable mobile data, managers were spending their lives trying to negotiate data roaming deals with mobile network operators in different countries. And, even then, service quality was a constant concern.
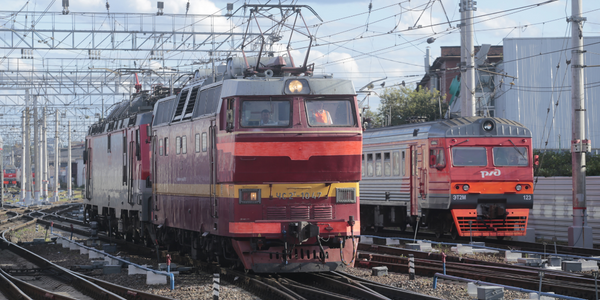
Case Study
Digitize Railway with Deutsche Bahn
To reduce maintenance costs and delay-causing failures for Deutsche Bahn. They need manual measurements by a position measurement system based on custom-made MEMS sensor clusters, which allow autonomous and continuous monitoring with wireless data transmission and long battery. They were looking for data pre-processing solution in the sensor and machine learning algorithms in the cloud so as to detect critical wear.
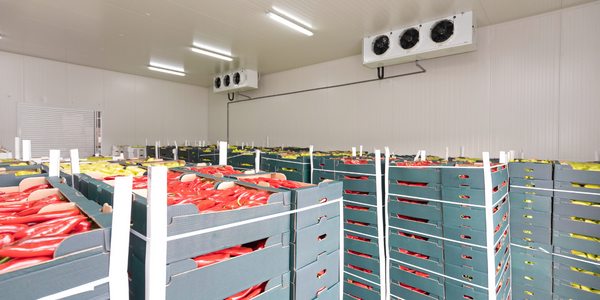
Case Study
Cold Chain Transportation and Refrigerated Fleet Management System
1) Create a digital connected transportation solution to retrofit cold chain trailers with real-time tracking and controls. 2) Prevent multi-million dollar losses due to theft or spoilage. 3) Deliver a digital chain-of-custody solution for door to door load monitoring and security. 4) Provide a trusted multi-fleet solution in a single application with granular data and access controls.
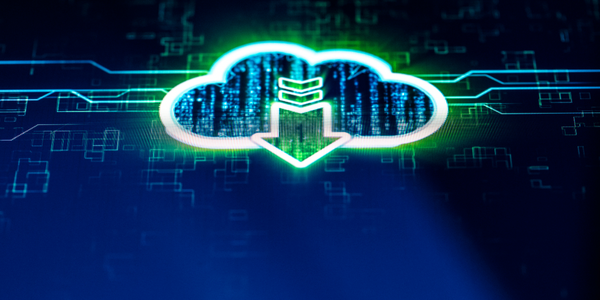
Case Study
3M Gains Real-Time Insight with Cloud Solution
The company has a long track record of innovative technology solutions. For example, 3M helps its customers optimize parking operations by automating fee collection and other processes. To improve support for this rapidly expanding segment, 3M needed to automate its own data collection and reporting. The company had recently purchased the assets of parking, tolling, and automatic license plate reader businesses, and required better insight into these acquisitions. Chad Reed, Global Business Manager for 3M Parking Systems, says, “With thousands of installations across the world, we couldn’t keep track of our software and hardware deployments, which made it difficult to understand our market penetration.” 3M wanted a tracking application that sales staff could use to get real-time information about the type and location of 3M products in parking lots and garages. So that it could be used on-site with potential customers, the solution would have to provide access to data anytime, anywhere, and from an array of mobile devices. Jason Fox, Mobile Application Architect at 3M, upped the ante by volunteering to deliver the new app in one weekend. For Fox and his team, these requirements meant turning to the cloud instead of an on-premises datacenter. “My first thought was to go directly to the cloud because we needed to provide access not only to our salespeople, but to resellers who didn’t have access to our internal network,” says Fox. “The cloud just seemed like a logical choice.”
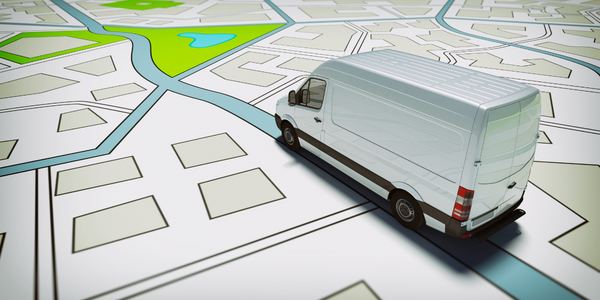
Case Study
IoT Based Asset Tracking System
The existing system used by the customer could only track a few thousand assets and was able to generate only a few standard set of reports. As the number of assets tracked grew exponentially, the system started to break at the seams. The Tracking devices were from different manufacturers following different protocols. There was no proper integration among the devices to send instant alerts. There are thousands of tracking devices spread across multiple geographies, that are moving. The configuration and troubleshooting of these devices incurred heavy costs, which was a logistics challenge. The existing system did not provide sophisticated Analytics, Business Intelligence and Insights from the data