Substantial data analysis improves gene-environmental correlation identification to help develop new treatment for multiple sclerosis
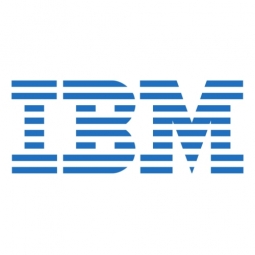
Customer Company Size
Large Corporate
Region
- America
Country
- United States
Product
- IBM® PureData™ System for Analytics
- IBM Business Partner Revolution Analytics
Tech Stack
- Data Analytics
- Data Warehousing
Implementation Scale
- Enterprise-wide Deployment
Impact Metrics
- Productivity Improvements
- Innovation Output
Technology Category
- Analytics & Modeling - Big Data Analytics
- Analytics & Modeling - Data Mining
Applicable Industries
- Education
- Healthcare & Hospitals
Applicable Functions
- Product Research & Development
Use Cases
- Predictive Maintenance
- Predictive Quality Analytics
Services
- Data Science Services
About The Customer
Founded in 1846, the State University of New York (SUNY) at Buffalo is the flagship institution in the State University of New York system. It is the largest and most comprehensive campus in the 64-campus SUNY system and home to one of the leading multiple sclerosis (MS) research centers in the world. University at Buffalo offers more than 100 undergraduate degrees, 205 master’s degrees, 84 doctoral degrees and 10 professional degrees.
The Challenge
As one of the leading multiple sclerosis (MS) research centers in the world, researchers at University at Buffalo wanted to identify and understand environmental factors that may contribute to MS. However, gene-environmental research presented researchers with enormous volumes of data for which they needed high-performance processing power and speed to make meaningful, publishable discoveries. A major challenge in gene interaction research is analyzing the explosions of immense data sets at a speed that will help save lives.
The Solution
University at Buffalo researchers use a powerful solution that combines data warehouse and analytic capabilities to handle the exponential growth rate of genetic variations involved in breakthrough data-mining methods for gene interaction in disease discovery for MS. The solution helps enable researchers to use new algorithms and analyze volumes of data that could number in the quintillions, which was previously impossible, allowing them to examine more than 2,000 genetic and environmental factors that may contribute to the development and progression of MS. For example, researchers can analyze vitamin D metabolites’ protective associations with disability and brain atrophy in MS and its possible correlation to why MS is more common in northern latitudes and less common toward the equator. And in turn, new insights can help develop therapeutic and prevention strategies for treating and managing MS.
Operational Impact
Quantitative Benefit
Case Study missing?
Start adding your own!
Register with your work email and create a new case study profile for your business.
Related Case Studies.
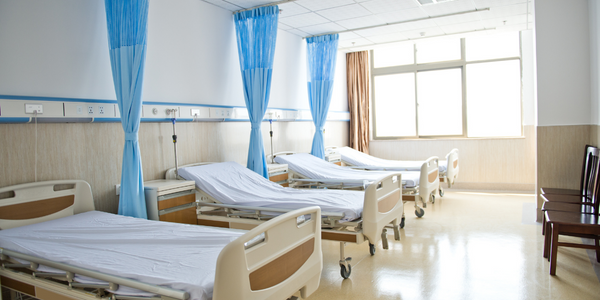
Case Study
Hospital Inventory Management
The hospital supply chain team is responsible for ensuring that the right medical supplies are readily available to clinicians when and where needed, and to do so in the most efficient manner possible. However, many of the systems and processes in use at the cancer center for supply chain management were not best suited to support these goals. Barcoding technology, a commonly used method for inventory management of medical supplies, is labor intensive, time consuming, does not provide real-time visibility into inventory levels and can be prone to error. Consequently, the lack of accurate and real-time visibility into inventory levels across multiple supply rooms in multiple hospital facilities creates additional inefficiency in the system causing over-ordering, hoarding, and wasted supplies. Other sources of waste and cost were also identified as candidates for improvement. Existing systems and processes did not provide adequate security for high-cost inventory within the hospital, which was another driver of cost. A lack of visibility into expiration dates for supplies resulted in supplies being wasted due to past expiry dates. Storage of supplies was also a key consideration given the location of the cancer center’s facilities in a dense urban setting, where space is always at a premium. In order to address the challenges outlined above, the hospital sought a solution that would provide real-time inventory information with high levels of accuracy, reduce the level of manual effort required and enable data driven decision making to ensure that the right supplies were readily available to clinicians in the right location at the right time.
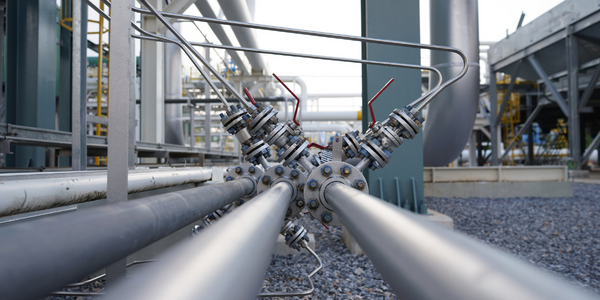
Case Study
Gas Pipeline Monitoring System for Hospitals
This system integrator focuses on providing centralized gas pipeline monitoring systems for hospitals. The service they provide makes it possible for hospitals to reduce both maintenance and labor costs. Since hospitals may not have an existing network suitable for this type of system, GPRS communication provides an easy and ready-to-use solution for remote, distributed monitoring systems System Requirements - GPRS communication - Seamless connection with SCADA software - Simple, front-end control capability - Expandable I/O channels - Combine AI, DI, and DO channels
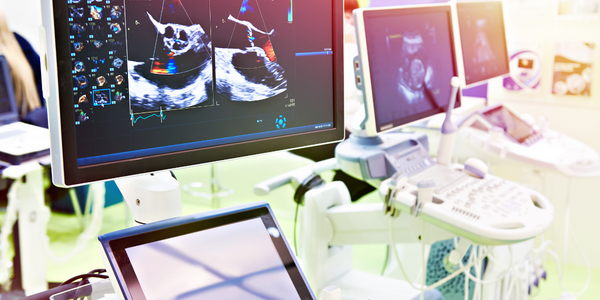
Case Study
Driving Digital Transformations for Vitro Diagnostic Medical Devices
Diagnostic devices play a vital role in helping to improve healthcare delivery. In fact, an estimated 60 percent of the world’s medical decisions are made with support from in vitrodiagnostics (IVD) solutions, such as those provided by Roche Diagnostics, an industry leader. As the demand for medical diagnostic services grows rapidly in hospitals and clinics across China, so does the market for IVD solutions. In addition, the typically high cost of these diagnostic devices means that comprehensive post-sales services are needed. Wanteed to improve three portions of thr IVD:1. Remotely monitor and manage IVD devices as fixed assets.2. Optimizing device availability with predictive maintenance.3. Recommending the best IVD solution for a customer’s needs.
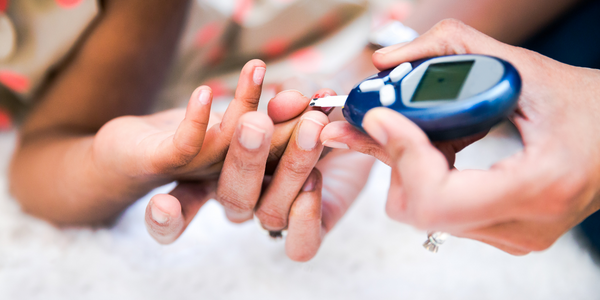
Case Study
HaemoCloud Global Blood Management System
1) Deliver a connected digital product system to protect and increase the differentiated value of Haemonetics blood and plasma solutions. 2) Improve patient outcomes by increasing the efficiency of blood supply flows. 3) Navigate and satisfy a complex web of global regulatory compliance requirements. 4) Reduce costly and labor-intensive maintenance procedures.
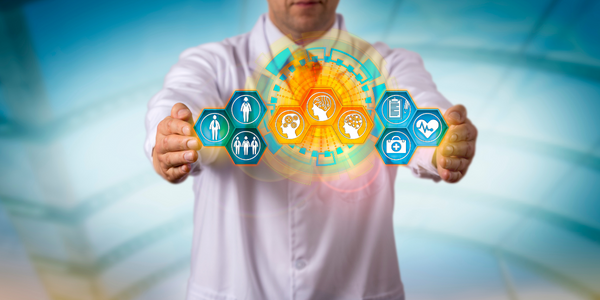
Case Study
Harnessing real-time data to give a holistic picture of patient health
Every day, vast quantities of data are collected about patients as they pass through health service organizations—from operational data such as treatment history and medications to physiological data captured by medical devices. The insights hidden within this treasure trove of data can be used to support more personalized treatments, more accurate diagnosis and more advanced preparative care. But since the information is generated faster than most organizations can consume it, unlocking the power of this big data can be a struggle. This type of predictive approach not only improves patient care—it also helps to reduce costs, because in the healthcare industry, prevention is almost always more cost-effective than treatment. However, collecting, analyzing and presenting these data-streams in a way that clinicians can easily understand can pose a significant technical challenge.