Company Size
200-1,000
Region
- America
Country
- United States
Product
- Sift
Tech Stack
- Machine Learning
Implementation Scale
- Enterprise-wide Deployment
Impact Metrics
- Cost Savings
- Productivity Improvements
Technology Category
- Analytics & Modeling - Machine Learning
Applicable Industries
- Retail
Applicable Functions
- Sales & Marketing
Use Cases
- Fraud Detection
Services
- Data Science Services
About The Customer
StackCommerce is the leading native commerce platform for online publishers, communities, and brands. They power deal stores for the world’s top tech and lifestyle publishers by offering curated product recommendations tailored to each client’s audience. A fast-growing business in a thriving market, StackCommerce has more than 1,500 vendors offering products and services to over 200 million monthly users across more than 750 publishers’ websites. As part of their service, StackCommerce handles fraud management for any orders placed on their platform.
The Challenge
StackCommerce, a leading native commerce platform, was dealing with a significant amount of fraud involving purchases made using stolen credit cards. The most impactful type of fraud was the loss of digital goods that are distributed instantly. This not only hurt cardholders but also the merchants. StackCommerce needed to stop these transactions as quickly as possible and sought a solution that could prevent them in the first place. They were using a legacy, rules-based solution that didn’t include any machine learning. As the company’s order volume grew, they discovered the shortcomings of rules-based systems: they don’t learn and they don’t scale. The team found themselves reviewing hundreds – or even thousands – of orders per day, and fraud review became unmanageable.
The Solution
StackCommerce began looking for a tool they could confidently rely on to prevent fraud, and which also had automation capabilities. After extensive online research – and a recommendation by their payment gateway, Stripe – they landed on Sift. Using Sift’s extensive online documentation, they were able to get up and running in less than two weeks. The team saw accurate results immediately, but the results were even more striking after they trained their machine learning model by labeling users. The StackCommerce team uses Lists to efficiently manage their fraud review process, making instant decisions or flagging orders for additional verification. They also use Sift’s automation tools – Formulas and Actions – to automate fraud decisions, saving even more of the team’s precious time.
Operational Impact
Quantitative Benefit
Case Study missing?
Start adding your own!
Register with your work email and create a new case study profile for your business.
Related Case Studies.
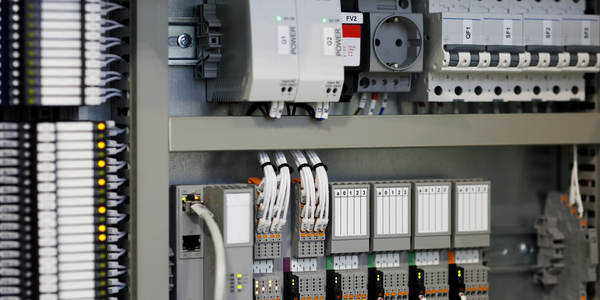
Case Study
Improving Production Line Efficiency with Ethernet Micro RTU Controller
Moxa was asked to provide a connectivity solution for one of the world's leading cosmetics companies. This multinational corporation, with retail presence in 130 countries, 23 global braches, and over 66,000 employees, sought to improve the efficiency of their production process by migrating from manual monitoring to an automatic productivity monitoring system. The production line was being monitored by ABB Real-TPI, a factory information system that offers data collection and analysis to improve plant efficiency. Due to software limitations, the customer needed an OPC server and a corresponding I/O solution to collect data from additional sensor devices for the Real-TPI system. The goal is to enable the factory information system to more thoroughly collect data from every corner of the production line. This will improve its ability to measure Overall Equipment Effectiveness (OEE) and translate into increased production efficiencies. System Requirements • Instant status updates while still consuming minimal bandwidth to relieve strain on limited factory networks • Interoperable with ABB Real-TPI • Small form factor appropriate for deployment where space is scarce • Remote software management and configuration to simplify operations
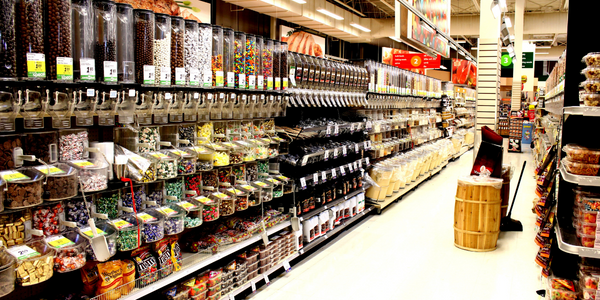
Case Study
How Sirqul’s IoT Platform is Crafting Carrefour’s New In-Store Experiences
Carrefour Taiwan’s goal is to be completely digital by end of 2018. Out-dated manual methods for analysis and assumptions limited Carrefour’s ability to change the customer experience and were void of real-time decision-making capabilities. Rather than relying solely on sales data, assumptions, and disparate systems, Carrefour Taiwan’s CEO led an initiative to find a connected IoT solution that could give the team the ability to make real-time changes and more informed decisions. Prior to implementing, Carrefour struggled to address their conversion rates and did not have the proper insights into the customer decision-making process nor how to make an immediate impact without losing customer confidence.
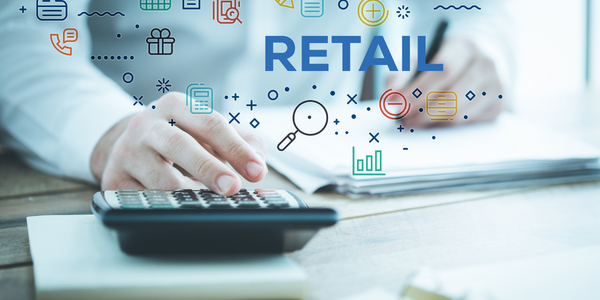
Case Study
Digital Retail Security Solutions
Sennco wanted to help its retail customers increase sales and profits by developing an innovative alarm system as opposed to conventional connected alarms that are permanently tethered to display products. These traditional security systems were cumbersome and intrusive to the customer shopping experience. Additionally, they provided no useful data or analytics.
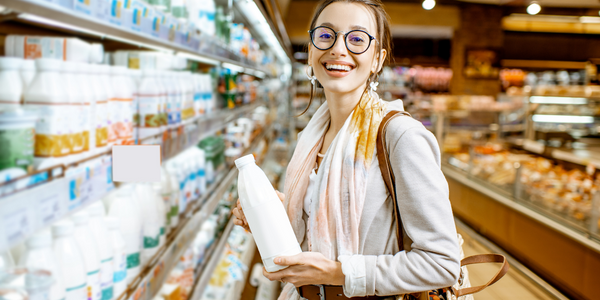
Case Study
Ensures Cold Milk in Your Supermarket
As of 2014, AK-Centralen has over 1,500 Danish supermarkets equipped, and utilizes 16 operators, and is open 24 hours a day, 365 days a year. AK-Centralen needed the ability to monitor the cooling alarms from around the country, 24 hours a day, 365 days a year. Each and every time the door to a milk cooler or a freezer does not close properly, an alarm goes off on a computer screen in a control building in southwestern Odense. This type of alarm will go off approximately 140,000 times per year, equating to roughly 400 alarms in a 24-hour period. Should an alarm go off, then there is only a limited amount of time to act before dairy products or frozen pizza must be disposed of, and this type of waste can quickly start to cost a supermarket a great deal of money.
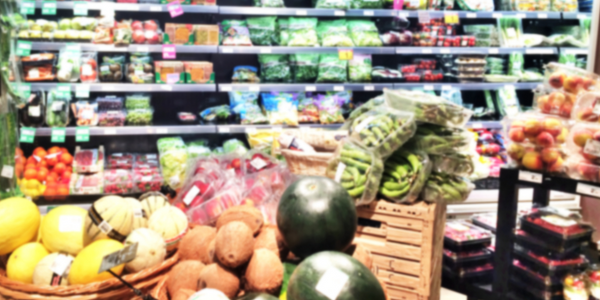
Case Study
Supermarket Energy Savings
The client had previously deployed a one-meter-per-store monitoring program. Given the manner in which energy consumption changes with external temperature, hour of the day, day of week and month of year, a single meter solution lacked the ability to detect the difference between a true problem and a changing store environment. Most importantly, a single meter solution could never identify root cause of energy consumption changes. This approach never reduced the number of truck-rolls or man-hours required to find and resolve issues.