Technology Category
- Analytics & Modeling - Big Data Analytics
- Application Infrastructure & Middleware - Database Management & Storage
Applicable Industries
- Buildings
- Construction & Infrastructure
Applicable Functions
- Sales & Marketing
Use Cases
- Construction Management
- Time Sensitive Networking
Services
- Data Science Services
- System Integration
About The Customer
Postman is an API collaboration platform that aims to simplify and expedite API processes. The company, which started as a side project six years ago, has grown to become one of India's latest unicorns with a valuation of $5.6 billion. Postman's platform is used by over 17 million people from 500,000 companies globally. The company has a 400-member team spread across two offices in Bangalore, one in San Francisco, and distributed remotely across four continents. The data team, which is based in Bangalore, consists of 25 members divided into two sub-teams: the Data Engineering Team and the Data Science Team.
The Challenge
Postman, an API collaboration platform, experienced rapid growth, with its valuation reaching $5.6 billion and its user base expanding to over 17 million people from 500,000 companies globally. However, the company's data team was not growing at the same pace. In April 2020, the data team consisted of only six or seven people. Over the next year, the team expanded by 4-5x to 25 people. This rapid growth presented challenges in terms of onboarding new hires, handling requests from the rest of the company, and planning their work. The data team was also grappling with the decision between a centralized and decentralized team structure, with the former leading to conflicting data systems and metrics. Additionally, the team faced difficulties in prioritizing work and allocating projects fairly among team members.
The Solution
To address these challenges, Postman's data team implemented several strategies. They moved towards a more centralized team structure, hiring analysts into the Data Science Team and training them centrally. After a several month onboarding process, some new hires would move to more data-mature teams as embedded or decentralized analysts. The team also introduced a hierarchy to distinguish between different levels of data analysts. To manage work prioritization, they implemented a ticketing system on Jira, where anyone in the organization could create a ticket detailing their data request. The team would then follow up on each ticket, gathering more information and assigning each ticket to a sprint based on its priority and impact. To allocate projects, the team introduced weekly Issue Grooming sessions, where they would assess and assign new tasks. The team also adopted the sprint methodology, breaking down projects into smaller tasks and conducting sprint retrospectives to continuously improve their processes.
Operational Impact
Quantitative Benefit
Case Study missing?
Start adding your own!
Register with your work email and create a new case study profile for your business.
Related Case Studies.

Case Study
Energy Saving & Power Monitoring System
Recently a university in Taiwan was experiencing dramatic power usage increases due to its growing number of campus buildings and students. Aiming to analyze their power consumption and increase their power efficiency across 52 buildings, the university wanted to build a power management system utilizing web-based hardware and software. With these goals in mind, they contacted Advantech to help them develop their system and provide them with the means to save energy in the years to come.

Case Study
IoT System for Tunnel Construction
The Zenitaka Corporation ('Zenitaka') has two major business areas: its architectural business focuses on structures such as government buildings, office buildings, and commercial facilities, while its civil engineering business is targeted at structures such as tunnels, bridges and dams. Within these areas, there presented two issues that have always persisted in regard to the construction of mountain tunnels. These issues are 'improving safety" and "reducing energy consumption". Mountain tunnels construction requires a massive amount of electricity. This is because there are many kinds of electrical equipment being used day and night, including construction machinery, construction lighting, and ventilating fan. Despite this, the amount of power consumption is generally not tightly managed. In many cases, the exact amount of power consumption is only ascertained when the bill from the power company becomes available. Sometimes, corporations install demand-monitoring equipment to help curb the maximum power demanded. However, even in these cases, the devices only allow the total volume of power consumption to be ascertained, or they may issue warnings to prevent the contracted volume of power from being exceeded. In order to tackle the issue of reducing power consumption, it was first necessary to obtain an accurate breakdown of how much power was being used in each particular area. In other words, we needed to be able to visualize the amount of power being consumed. Safety, was also not being managed very rigorously. Even now, tunnel construction sites often use a 'name label' system for managing entry into the work site. Specifically, red labels with white reverse sides that bear the workers' names on both sides are displayed at the tunnel work site entrance. The workers themselves then flip the name label to the appropriate side when entering or exiting from the work site to indicate whether or not they are working inside the tunnel at any given time. If a worker forgets to flip his or her name label when entering or exiting from the tunnel, management cannot be performed effectively. In order to tackle the challenges mentioned above, Zenitaka decided to build a system that could improve the safety of tunnel construction as well as reduce the amount of power consumed. In other words, this new system would facilitate a clear picture of which workers were working in each location at the mountain tunnel construction site, as well as which processes were being carried out at those respective locations at any given time. The system would maintain the safety of all workers while also carefully controlling the electrical equipment to reduce unnecessary power consumption. Having decided on the concept, our next concern was whether there existed any kind of robust hardware that would not break down at the construction work site, that could move freely in response to changes in the working environment, and that could accurately detect workers and vehicles using radio frequency identification (RFID). Given that this system would involve many components that were new to Zenitaka, we decided to enlist the cooperation of E.I.Sol Co., Ltd. ('E.I.Sol') as our joint development partner, as they had provided us with a highly practical proposal.

Case Study
Intelligent Building Automation System and Energy Saving Solution
One of the most difficult problems facing the world is conserving energy in buildings. However, it is not easy to have a cost-effective solution to reduce energy usage in a building. One solution for saving energy is to implement an intelligent building automation system (BAS) which can be controlled according to its schedule. In Indonesia a large university with a five floor building and 22 classrooms wanted to save the amount of energy being used.

Case Study
Powering Smart Home Automation solutions with IoT for Energy conservation
Many industry leaders that offer Smart Energy Management products & solutions face challenges including:How to build a scalable platform that can automatically scale-up to on-board ‘n’ number of Smart home devicesData security, solution availability, and reliability are the other critical factors to deal withHow to create a robust common IoT platform that handles any kind of smart devicesHow to enable data management capabilities that would help in intelligent decision-making
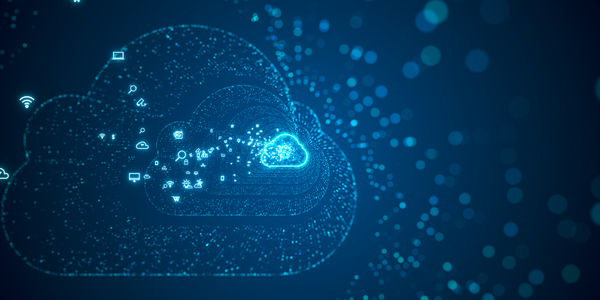
Case Study
Splunk Partnership Ties Together Big Data & IoT Services
Splunk was faced with the need to meet emerging customer demands for interfacing IoT projects to its suite of services. The company required an IoT partner that would be able to easily and quickly integrate with its Splunk Enterprise platform, rather than allocating development resources and time to building out an IoT interface and application platform.