Put a Label on It
Company Size
1,000+
Region
- Europe
Country
- Germany
Product
- understand.ai
- dSPACE
Tech Stack
- Neural Networks
- GPU-based Computer Clusters
- 3-D Annotation Tools
Implementation Scale
- Enterprise-wide Deployment
Impact Metrics
- Customer Satisfaction
- Innovation Output
- Productivity Improvements
Technology Category
- Analytics & Modeling - Machine Learning
- Analytics & Modeling - Predictive Analytics
- Application Infrastructure & Middleware - Data Visualization
Applicable Industries
- Automotive
- Software
Applicable Functions
- Product Research & Development
- Quality Assurance
Use Cases
- Machine Condition Monitoring
- Predictive Maintenance
Services
- Data Science Services
- System Integration
- Training
About The Customer
Bosch is a leading global supplier of technology and services, operating in various sectors including automotive, industrial technology, consumer goods, and energy and building technology. With a strong focus on innovation and quality, Bosch aims to enhance the quality of life with solutions that are both innovative and beneficial. The company employs over 400,000 associates worldwide and is known for its pioneering work in the field of autonomous driving. Bosch collaborates with various partners and leverages cutting-edge technologies to develop reliable and efficient systems for autonomous vehicles. Their commitment to research and development is evident in their continuous efforts to explore new technologies and methodologies to improve safety and efficiency in mobility solutions.
The Challenge
New innovative concepts for mobility, such as highly automated or autonomous driving, place enormous demands on the safety and reliability of technical systems. The efficient development of particularly reliable control systems for autonomous driving up to SAE Level 5 requires the use of suitable technologies. Conventional control-based approaches compete with the capabilities of trained neural networks, which are particularly suitable for processing vast amounts of data from high-resolution sensors. The first step is to identify potential application areas for AI along the entire processing chain from perception to situation analysis and behavior planning. Promising methods from machine learning must be evaluated, focusing on multimodal perception, i.e., the environment perception of a vehicle with merged data from video, radar, and lidar sensors.
The Solution
The raw data from sensors installed in vehicles, recorded during real drives, is used as training data. The traffic environments for the recordings are diverse, covering highways, country roads, urban areas, and various traffic scenarios. This diversity is achieved by defining ideal types and characteristics of routes and selecting tracks that match these definitions. Neural networks learn by means of positive and negative examples, a process called supervised learning. The tasks and solutions, typically raw sensor data and detected objects, are required to identify correct results. The solutions are made available by markings (labels/annotations) in the form of reference data. The anonymized data is transferred to understand.ai, a dSPACE group company specializing in labeling automation. Bosch and understand.ai agreed on precise quality goals to ensure successful training. Annotation was performed with data from lidar sensors, marked with high-precision bounding boxes in the 3-D point clouds. Camera sensor data was used for plausibility checks. An iterative approach with feedback loops ensured the required quality level early and continuously.
Operational Impact
Quantitative Benefit
Case Study missing?
Start adding your own!
Register with your work email and create a new case study profile for your business.
Related Case Studies.
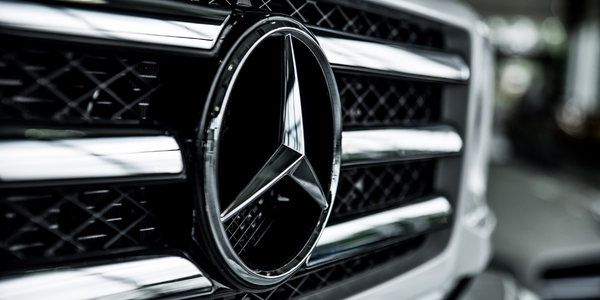
Case Study
Integral Plant Maintenance
Mercedes-Benz and his partner GAZ chose Siemens to be its maintenance partner at a new engine plant in Yaroslavl, Russia. The new plant offers a capacity to manufacture diesel engines for the Russian market, for locally produced Sprinter Classic. In addition to engines for the local market, the Yaroslavl plant will also produce spare parts. Mercedes-Benz Russia and his partner needed a service partner in order to ensure the operation of these lines in a maintenance partnership arrangement. The challenges included coordinating the entire maintenance management operation, in particular inspections, corrective and predictive maintenance activities, and the optimizing spare parts management. Siemens developed a customized maintenance solution that includes all electronic and mechanical maintenance activities (Integral Plant Maintenance).
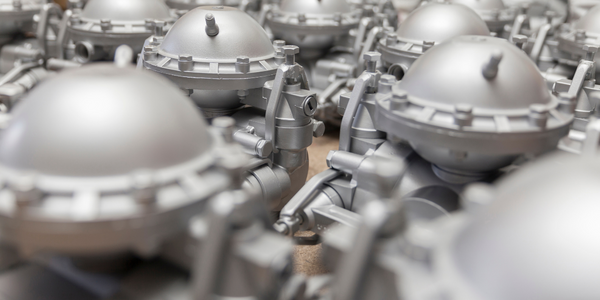
Case Study
Monitoring of Pressure Pumps in Automotive Industry
A large German/American producer of auto parts uses high-pressure pumps to deburr machined parts as a part of its production and quality check process. They decided to monitor these pumps to make sure they work properly and that they can see any indications leading to a potential failure before it affects their process.