Optimizing Smelting and Refining Equipment Reliability with Prescriptive Analytics
Company Size
1,000+
Product
- Aspen Mtell
Tech Stack
- Machine Learning
- Predictive Analytics
Implementation Scale
- Enterprise-wide Deployment
Impact Metrics
- Cost Savings
- Customer Satisfaction
- Productivity Improvements
Technology Category
- Analytics & Modeling - Machine Learning
- Analytics & Modeling - Predictive Analytics
Applicable Industries
- Metals
- Mining
Applicable Functions
- Discrete Manufacturing
- Maintenance
Use Cases
- Machine Condition Monitoring
- Predictive Maintenance
Services
- Data Science Services
About The Customer
The customer is one of the world’s largest fully integrated zinc and lead smelting and refining complexes. As a producer of refined zinc and lead, a variety of precious and specialty metals, chemicals and fertilizer products, their team’s success is based on improving best practices, optimizing efficient processes, reducing failures and increasing the bottom line. They wanted to improve their metallurgical operations and recognized an opportunity to improve preventative maintenance by using information from their process signal historian. They also wanted a solution that could help as the company developed a comprehensive approach to strengthen environmental, employee and community safeguards.
The Challenge
One of the world’s largest fully integrated zinc and lead smelting and refining complexes wanted to improve their metallurgical operations. The team recognized they had an opportunity to improve preventative maintenance by using information from their process signal historian. In addition, they wanted a solution that could help as the company developed a comprehensive approach to strengthen environmental, employee and community safeguards. The operations group’s reliability team needed a technology to track, detect and prevent equipment failures.
The Solution
The customer utilized Aspen Mtell machine learning to track and predict equipment failure as well as determine the precise process signature leading to a failure. Mtell has the ability to read process signals and calculate how much runtime a piece of equipment has left, and even automatically file a work order. The agent within Mtell provided guidance of a time-to-failure of roughly 40 days on a process crucial pump. The maintenance and reliability team acted and performed a detailed SWOT analysis to determine the best course of action based not only on the tool’s guidance, but on the site’s production forecast as well.
Operational Impact
Quantitative Benefit
Case Study missing?
Start adding your own!
Register with your work email and create a new case study profile for your business.
Related Case Studies.
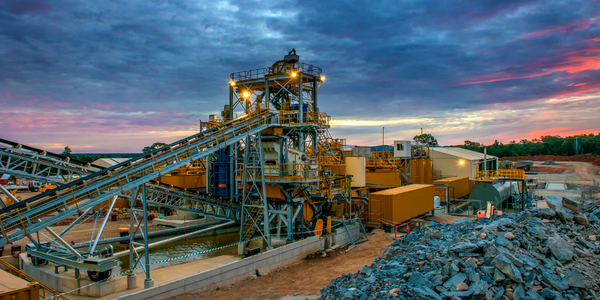
Case Study
Goldcorp: Internet of Things Enables the Mine of the Future
Goldcorp is committed to responsible mining practices and maintaining maximum safety for its workers. At the same time, the firm is constantly exploring ways to improve the efficiency of its operations, extend the life of its assets, and control costs. Goldcorp needed technology that can maximize production efficiency by tracking all mining operations, keep employees safe with remote operations and monitoring of hazardous work areas and control production costs through better asset and site management.
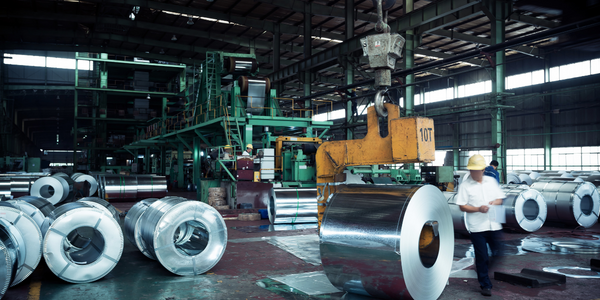
Case Study
KSP Steel Decentralized Control Room
While on-site in Pavlodar, Kazakhstan, the DAQRI team of Business Development and Solutions Architecture personnel worked closely with KSP Steel’s production leadership to understand the steel production process, operational challenges, and worker pain points.
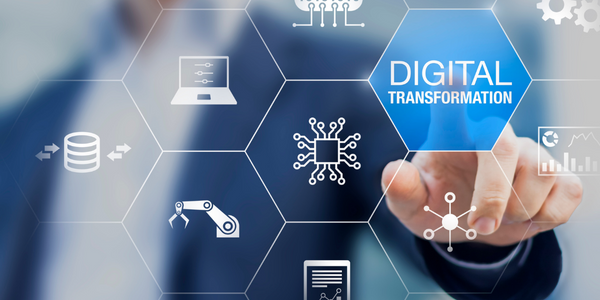
Case Study
Bluescope Steel on Path to Digitally Transform Operations and IT
Increasing competition and fluctuations in the construction market prompted BlueScope Steel to look toward digital transformation of its four businesses, including modern core applications and IT infrastructure. BlueScope needed to modernize its infrastructure and adopt new technologies to improve operations and supply chain efficiency while maintaining and updating an aging application portfolio.
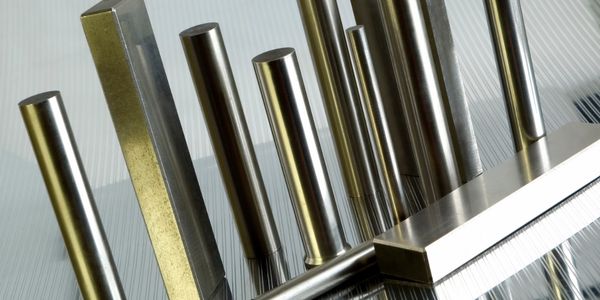
Case Study
RobotStudio Case Study: Benteler Automobiltechnik
Benteler has a small pipe business area for which they produce fuel lines and coolant lines made of aluminum for Porsche and other car manufacturers. One of the problems in production was that when Benteler added new products, production had too much downtime.
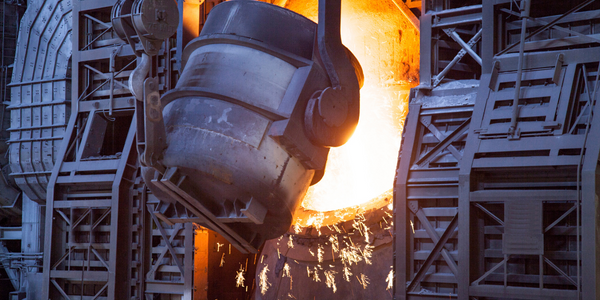
Case Study
Continuous Casting Machines in a Steel Factory
With a very broad range of applications, steel is an important material and has been developed into the most extensive alloy in the engineering world. Since delivering high quality is absolutely crucial for steel plants, ensuring maximum productivity and the best quality production are the keys to competitiveness in the steel industry. Additionally, working conditions in steel factories are not suitable for workers to stay in for long periods of time, so manufactures usually adopt various machines to complete the steel production processes. However, the precision of these machines is often overestimated and the lack of flexibility also makes supervisors unable to adjust operating procedures. A renowned steel factory in Asia planned to improve its Distributed Control System (DCS) of furnaces as well as addressing the problem of insufficient accuracy. However, most well-known international equipment suppliers can not provide a satisfactory solution and local maintenance because the project needed new technologies to more accurately control equipment operations. By implementing Advantech’s automated monitoring and control solution, steel factories can not only improve the manufacturing processes but can also allow users to add additional functions to the existing system so as to make sure the operation runs at high efficiency.
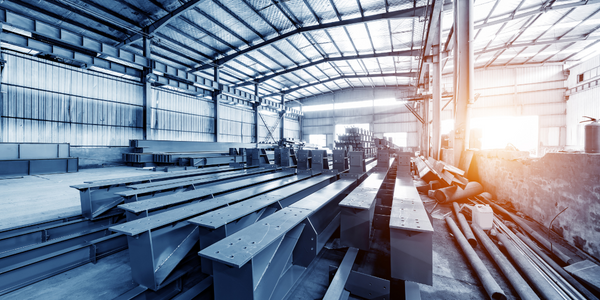
Case Study
Automated Predicitive Analytics For Steel/Metals Industry
Asset to be monitored: Wire Compactor that produces Steel RebarCustomer Faced The Following Challenges:Dependent upon machine uptime.Pressure cylinders within the compactor fail to control compression and speed causing problems in binding the coil.Equipment failure occurs in the final stage of production causing the entire line to stop, can you say bottleneck?Critical asset unequipped with sensors to produce data.