Technology Category
- Analytics & Modeling - Computer Vision Software
- Analytics & Modeling - Machine Learning
Applicable Industries
- Agriculture
- Education
Applicable Functions
- Product Research & Development
- Quality Assurance
Use Cases
- Computer Vision
- Visual Quality Detection
Services
- Testing & Certification
- Training
About The Customer
The customer in this case study is the BioMedIA research group at Imperial College London and the iFind Project. They are involved in medical research and were looking for ways to speed up the process of creating high-quality training data for machine learning use cases in the medical field. They partnered with Labelbox, a leading training data platform for machine learning applications, to conduct an experiment that challenged the assumption that only medical experts can provide quality annotations for clinical deep learning models.
The Challenge
Creating high-quality training data for machine learning (ML) use cases can be expensive and time-consuming, especially for specialized fields that require domain experts to review and label the data. This is particularly true in the medical field, where doctors are often required to meticulously label or review training data. This process can be arduous and costly, slowing down the development of potentially life-saving algorithms. The challenge was to find a way to create high-quality training data with less involvement from physicians, thereby reducing costs and speeding up the development process.
The Solution
A study conducted by researchers from Imperial College London’s BioMedIA research group and the iFind Project, in partnership with Labelbox, challenged the assumption that only medical experts can provide quality annotations for clinical deep learning models. The team conducted an experiment where minimally trained, novice labeling workforces were tasked with annotating fetal ultrasound imagery for signs of a specific congenital heart disease. The researchers then trained the same algorithm on two datasets: one labeled by the Labelbox workforces and one labeled by experts in medical imagery. The experiment found that these novice annotators could perform complex medical image segmentation tasks to a high standard, with their labels being similar in quality to those provided by experts. The researchers concluded that novice labeling teams can play a vital role in developing high-performing models in specialized medical use cases when employed in combination with existing methods to handle noisy annotations and choose the most informative annotations for the model.
Operational Impact
Quantitative Benefit
Case Study missing?
Start adding your own!
Register with your work email and create a new case study profile for your business.
Related Case Studies.
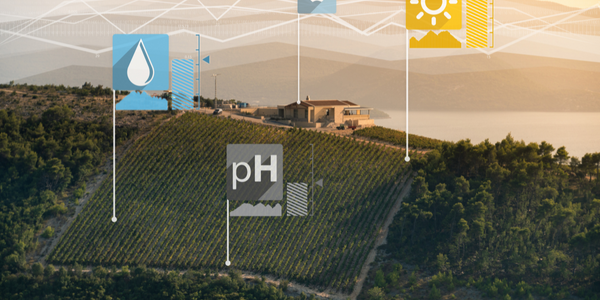
Case Study
Intelligent Farming with ThingWorx Analytics
Z Farms was facing three challenges: costly irrigation systems with water as a limited resource, narrow optimal ranges of soil moisture for growth with difficult maintenance and farm operators could not simply turn on irrigation systems like a faucet.
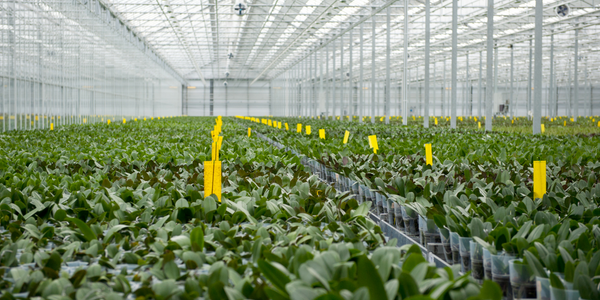
Case Study
Greenhouse Intelligent Monitoring and Control Solution
Farming Orchids is the most successful form of precision farming in Taiwan, and also the most exported flower. Orchids need a specific temperature and humidity conditions to grow and bloom, and its flowering time may not be in line with market demands, so the price collapses when there is overproduction. Therefore, some farmers began to import automated greenhouse control systems for breeding and forcing, which not only improves quality, but also effectively controls the production period and yield to ensure revenue. In 2012, an orchid farmer built a Forcing Greenhouse of about 200 pings (approximately 661 Square Meters) in Tainan, Taiwan. The system integrator adopted Advantech’s APAX-5000 series programmable automation controllers to build the control platform, coupled with Advantech WebAccess HMI/SCADA software, to achieve cloud monitoring. The staff of the orchid field can monitor important data anytime via smart phone, iPad, and other handheld devices, and control the growth and flowering conditions. System requirements: In the past, most environmental control systems of orchid greenhouses in Taiwan used PLCs (Programmable Logic Controller) with poorscalability and control, and could not be connected to the Internet formonitoring from the cloud. For advanced database analysis and networking capability, the PC platform must be adopted. Therefore, PAC Systems (Programmable Automation Controller) with both PLC programming capabilities andPC functions is a better choice.The environmental control of the Orchid greenhouse switches on and off devices like fan, shade net, cooling/heat pump, liquid flow control, water-cooling wall etc. It is controlled by a control panel of electric controllers, and is driven by a motor, to adjust the greenhouse temperature, humidity, and other environmental conditions to the set parameters.
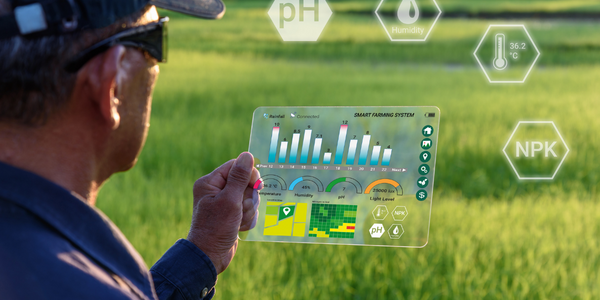
Case Study
Enabling Internet of Things Innovation in Agriculture
DigiBale, wanted to apply technology know-how and IP from implementations successfully to more agriculture sectors including cotton, forestry, sugarcane and cattle. However, farmers and growers still have worries about the connected technology.
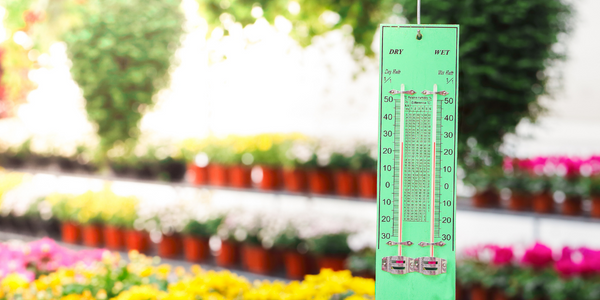
Case Study
Precision beekeeping with wireless temperature monitoring
Honeybees are insects of large economic value and provide a vital service to agriculture by pollinating a variety of crops. In addition, bees provide us with valuable products such as honey, beeswax, propolis, bee venom, etc. Monitoring of honeybee colony health, population, productivity, and environmental conditions affecting the colony health have always been exceedingly difficult tasks in apiculture. Research has shown that even small deviations (by more than 2°C) from the optimal temperatures have a significant influence on the development of the brood and the health of adult bees.