Multivariate Statistical Analysis Finds the Bad Actors in Out-of-Spec Batches
Product
- Aspen ProMV
Tech Stack
- Machine Learning
- Data Analysis
Implementation Scale
- Pilot projects
Impact Metrics
- Cost Savings
- Productivity Improvements
Technology Category
- Analytics & Modeling - Big Data Analytics
- Analytics & Modeling - Predictive Analytics
Applicable Industries
- Chemicals
Applicable Functions
- Discrete Manufacturing
- Quality Assurance
Use Cases
- Predictive Quality Analytics
- Process Control & Optimization
Services
- Data Science Services
About The Customer
The customer in this case study is a large producer of synthetic rubber. The company has been facing quality issues with its batch products, leading to significant revenue loss. The company often had to either reprocess the material or sell it at a lower price than expected due to these quality issues. The company was unable to determine the cause of these out-of-spec batches. The company has a long-standing relationship with AspenTech and uses a number of products from the aspenONE® Manufacturing and Supply Chain and Engineering suites.
The Challenge
A large producer of synthetic rubber had been having quality issues with its batch products. These quality issues were resulting in significant revenue loss, as the company often needed to either reprocess the material or sell it for a lower price than expected. The producer was unable to determine what was causing the batches to be out of spec. The company was investigating issues with a reactor process that brings together ingredients to manufacture synthetic rubber. There were multiple reactors that performed this process, but the Aspen ProMV project would focus on the production of one reactor.
The Solution
Aspen ProMV desktop batch model was developed to identify the bad actors in the off-spec batches. The customer provided five months of production data, representing 55 batches produced from this one reactor. Input variables included initial temperature, amount of catalyst and amount of other raw materials for each batch. Since this was a batch process, there was batch profile data (e.g., temperature, pressure, level, reactor agitator speeds, etc.) from the batch run. Quality variables measured at the end of each batch were also provided. There were three key quality variables that customer wanted to keep in control. The analysis was performed using Aspen ProMV desktop for batch. Aspen ProMV found several variables with very low variations and excluded them from the model.
Operational Impact
Case Study missing?
Start adding your own!
Register with your work email and create a new case study profile for your business.
Related Case Studies.
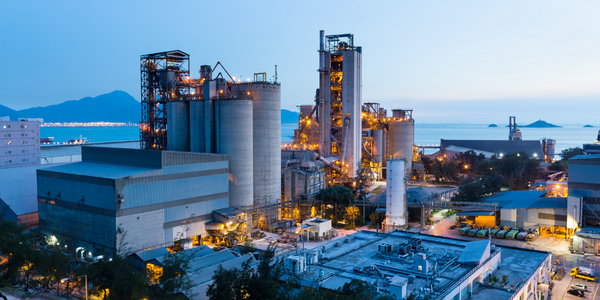
Case Study
Honeywell - Tata Chemicals Improves Data Accessibility with OneWireless
Tata was facing data accessibility challenges in the cement plant control room tapping signals from remote process control areas and other distant locations, including the gas scrubber. Tata needed a wireless solution to extend its control network securely to remote locations that would also provide seamless communication with existing control applications.

Case Study
Advanced Elastomer Systems Upgrades Production
In order to maintain its share of the international market for thermoplastic elastomers AES recently expanded its Florida plant by adding a new production line. While the existing lines were operating satisfactorily using a PROVOX distributed control system with traditional analog I/O, AES wanted advanced technology on the new line for greater economy, efficiency, and reliability. AES officials were anxious to get this line into production to meet incoming orders, but two hurricanes slowed construction.
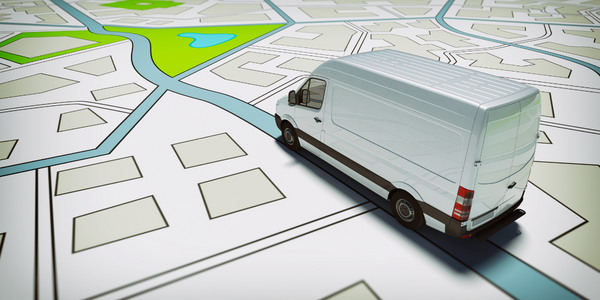
Case Study
Wireless GPS Tracking & Security Monitoring
Enhancing the security of hazardous freight and ensuring compliance with Homeland Security’s Transportation Security Administration mandate that all trains carrying chemicals capable of creating a toxic inhalation condition are equipped with on-board safety monitoring systems.
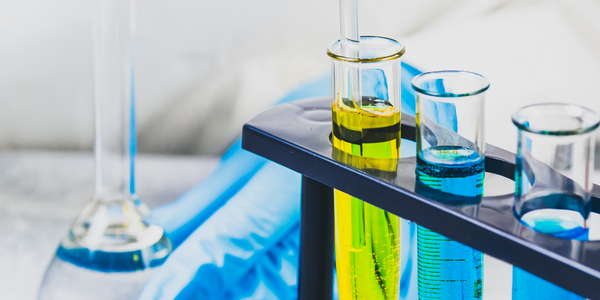
Case Study
Field Device Asset Management For Chemical Company in China
Chinese chemical subsidiary of multinational corporation serves customers throughout the world. Sales offices and research and technology centers are strategically located to provide rapid response to customer requests. Just two workers were assigned to maintain thousands of intelligent instruments in three production units, so they could do little more than react to device issues as they appeared. This costly maintenance method inevitably led to unexpected downtime when a critical instrument failed. Plant management recognized the need to change from reactive to predictive maintenance for all assets, including instruments and control valves, but help was needed in implementing such a technology-based initiative.
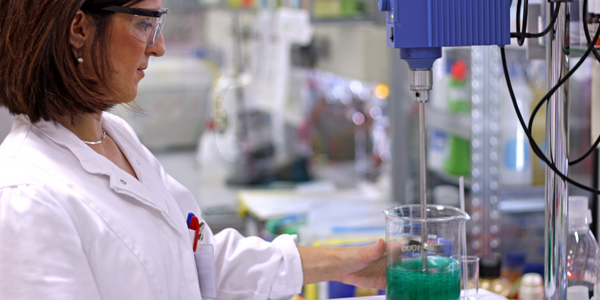
Case Study
Industrial Workforce Mobility for Improved Safety & Operations
Huntsman Corporation, a global manufacturer and marketer of differentiated chemicals, undertook an aggressive program to eliminate injuries, product defects, and environmental releases at their Port Neches facility. Termed “Project Zero”, this program required a completely mobile solution to empower operations and maintenance personnel to capture defects, track work progress and make process and safety related decisions in real-time.