Company Size
1,000+
Region
- Europe
Country
- United Kingdom
Product
- Run:ai's Platform
Tech Stack
- AI Hardware
- Deep Learning Training Models
- GPU Compute
Implementation Scale
- Enterprise-wide Deployment
Impact Metrics
- Innovation Output
- Productivity Improvements
Technology Category
- Analytics & Modeling - Machine Learning
- Application Infrastructure & Middleware - API Integration & Management
Applicable Industries
- Healthcare & Hospitals
Applicable Functions
- Product Research & Development
Use Cases
- Computer Vision
- Predictive Maintenance
Services
- Data Science Services
- System Integration
About The Customer
The London Medical Imaging & Artificial Intelligence Centre for Value Based Healthcare is a consortium of academic, healthcare and industry partners, led by King’s College London and based at St. Thomas’ Hospital. It uses medical images and electronic healthcare data held by the UK National Health Service to train sophisticated deep learning algorithms for computer vision and natural-language processing. These algorithms are used to create new tools for effective screening, faster diagnosis and personalized therapies, to improve patients’ health.
The Challenge
The London Medical Imaging & AI Centre for Value Based Healthcare was facing several challenges with its AI hardware. The total GPU utilization was below 30%, with significant idle periods for some GPUs despite demand from researchers. The system was overloaded on multiple occasions where more GPUs were needed for running jobs than were available. Poor visibility and scheduling led to delays and waste, with bigger experiments requiring a large number of GPUs sometimes unable to begin because smaller jobs using only a few GPUs were blocking them out of their resource requirements.
The Solution
The AI Centre implemented Run:ai's Platform to address these challenges. The platform increased GPU utilization by 110%, with resultant increases in experiment speed. Researchers ran more than 300 experiments in a 40-day period, compared to just 162 experiments that were run in a simulation of the same environment without Run:ai. By dynamically allocating pooled GPU to workloads, hardware resources were shared more efficiently. The platform also improved visibility with advanced monitoring and cluster management tools, allowing data scientists to see which GPU resources were not being used and dynamically adjust the size of their job to run on available capacity. The platform also enabled fair scheduling and guaranteed resources, allowing large ongoing workloads to use the optimal amount of GPU during low-demand times, and automatically allowing shorter, higher-priority workloads to run alongside.
Operational Impact
Quantitative Benefit
Case Study missing?
Start adding your own!
Register with your work email and create a new case study profile for your business.
Related Case Studies.
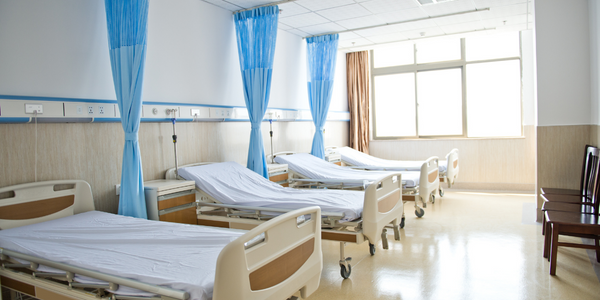
Case Study
Hospital Inventory Management
The hospital supply chain team is responsible for ensuring that the right medical supplies are readily available to clinicians when and where needed, and to do so in the most efficient manner possible. However, many of the systems and processes in use at the cancer center for supply chain management were not best suited to support these goals. Barcoding technology, a commonly used method for inventory management of medical supplies, is labor intensive, time consuming, does not provide real-time visibility into inventory levels and can be prone to error. Consequently, the lack of accurate and real-time visibility into inventory levels across multiple supply rooms in multiple hospital facilities creates additional inefficiency in the system causing over-ordering, hoarding, and wasted supplies. Other sources of waste and cost were also identified as candidates for improvement. Existing systems and processes did not provide adequate security for high-cost inventory within the hospital, which was another driver of cost. A lack of visibility into expiration dates for supplies resulted in supplies being wasted due to past expiry dates. Storage of supplies was also a key consideration given the location of the cancer center’s facilities in a dense urban setting, where space is always at a premium. In order to address the challenges outlined above, the hospital sought a solution that would provide real-time inventory information with high levels of accuracy, reduce the level of manual effort required and enable data driven decision making to ensure that the right supplies were readily available to clinicians in the right location at the right time.
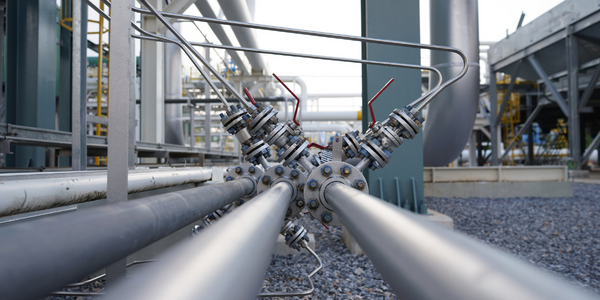
Case Study
Gas Pipeline Monitoring System for Hospitals
This system integrator focuses on providing centralized gas pipeline monitoring systems for hospitals. The service they provide makes it possible for hospitals to reduce both maintenance and labor costs. Since hospitals may not have an existing network suitable for this type of system, GPRS communication provides an easy and ready-to-use solution for remote, distributed monitoring systems System Requirements - GPRS communication - Seamless connection with SCADA software - Simple, front-end control capability - Expandable I/O channels - Combine AI, DI, and DO channels
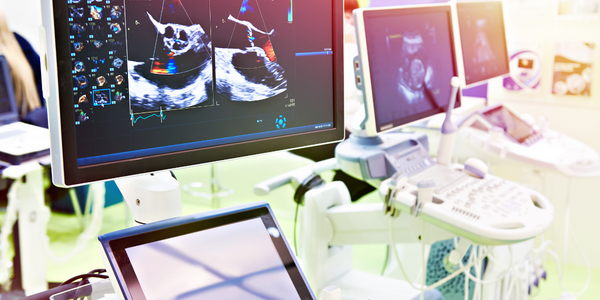
Case Study
Driving Digital Transformations for Vitro Diagnostic Medical Devices
Diagnostic devices play a vital role in helping to improve healthcare delivery. In fact, an estimated 60 percent of the world’s medical decisions are made with support from in vitrodiagnostics (IVD) solutions, such as those provided by Roche Diagnostics, an industry leader. As the demand for medical diagnostic services grows rapidly in hospitals and clinics across China, so does the market for IVD solutions. In addition, the typically high cost of these diagnostic devices means that comprehensive post-sales services are needed. Wanteed to improve three portions of thr IVD:1. Remotely monitor and manage IVD devices as fixed assets.2. Optimizing device availability with predictive maintenance.3. Recommending the best IVD solution for a customer’s needs.
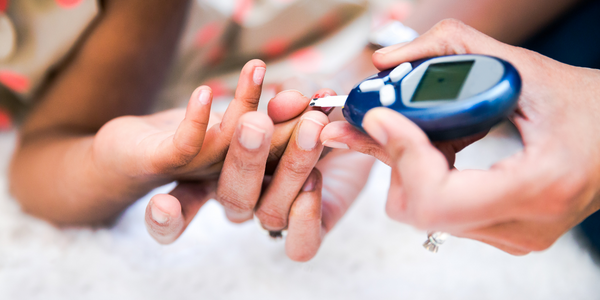
Case Study
HaemoCloud Global Blood Management System
1) Deliver a connected digital product system to protect and increase the differentiated value of Haemonetics blood and plasma solutions. 2) Improve patient outcomes by increasing the efficiency of blood supply flows. 3) Navigate and satisfy a complex web of global regulatory compliance requirements. 4) Reduce costly and labor-intensive maintenance procedures.
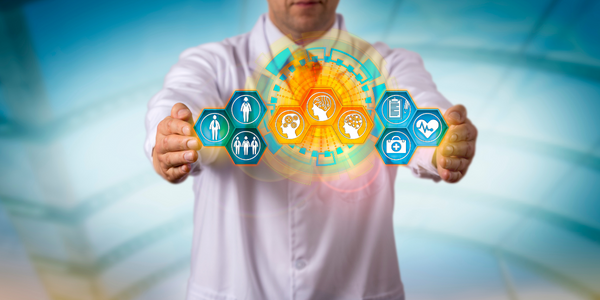
Case Study
Harnessing real-time data to give a holistic picture of patient health
Every day, vast quantities of data are collected about patients as they pass through health service organizations—from operational data such as treatment history and medications to physiological data captured by medical devices. The insights hidden within this treasure trove of data can be used to support more personalized treatments, more accurate diagnosis and more advanced preparative care. But since the information is generated faster than most organizations can consume it, unlocking the power of this big data can be a struggle. This type of predictive approach not only improves patient care—it also helps to reduce costs, because in the healthcare industry, prevention is almost always more cost-effective than treatment. However, collecting, analyzing and presenting these data-streams in a way that clinicians can easily understand can pose a significant technical challenge.