Customer Company Size
Large Corporate
Product
- Run:ai's Platform
Tech Stack
- Nvidia DGX servers
- GPU workstations
Implementation Scale
- Enterprise-wide Deployment
Impact Metrics
- Productivity Improvements
- Cost Savings
Technology Category
- Analytics & Modeling - Machine Learning
- Application Infrastructure & Middleware - API Integration & Management
Applicable Industries
- Software
- Telecommunications
Applicable Functions
- Product Research & Development
- Business Operation
Use Cases
- Predictive Maintenance
- Computer Vision
Services
- Data Science Services
- System Integration
About The Customer
The customer is a multinational company that is a world leader in facial recognition technologies. They provide AI services to many large enterprises, often in real-time. Accuracy, measured in terms of maximizing performance of camera resolution and FPS, density of faces, and field of view are critically important to the company and their customers. They have an on-premises environment with 24 Nvidia DGX servers and additional GPU workstations, and a team of 30 researchers spread across two continents.
The Challenge
The company, a world leader in facial recognition technologies, was facing several challenges with their GPU utilization. They were unable to successfully share resources across teams and projects due to static allocation of GPU resources, which led to bottlenecks and inaccessible infrastructure. The lack of visibility and management of available resources was slowing down their jobs. Despite the low utilization of existing hardware, visibility issues and bottlenecks made it seem like additional hardware was necessary, leading to increased costs. The company was considering an additional GPU investment with a planned hardware purchase cost of over $1 million dollars.
The Solution
The company implemented Run:ai's platform to address their challenges. The platform increased GPU utilization by moving teams from static, manual GPU allocations to pooled, dynamic resource sharing across the organization. It also increased productivity for the data science teams using hardware abstraction, simplified workflows, and automated GPU resource allocations. The platform provided visibility into the GPU cluster, its utilization, usage patterns, wait times, etc., allowing the company to better plan hardware spending. Furthermore, it accelerated training times, using automated, dynamic allocation of resources which enabled the data science teams to complete training processes significantly faster.
Operational Impact
Quantitative Benefit
Case Study missing?
Start adding your own!
Register with your work email and create a new case study profile for your business.
Related Case Studies.
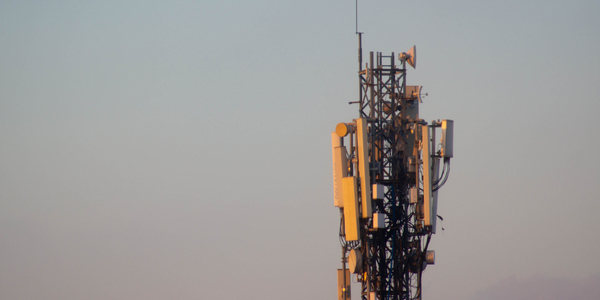
Case Study
Vodafone Hosted On AWS
Vodafone found that traffic for the applications peak during the four-month period when the international cricket season is at its height in Australia. During the 2011/2012 cricket season, 700,000 consumers downloaded the Cricket Live Australia application. Vodafone needed to be able to meet customer demand, but didn’t want to invest in additional resources that would be underutilized during cricket’s off-season.
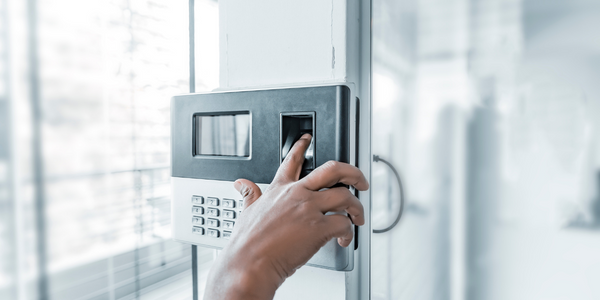
Case Study
SKT, Construction of Smart Office Environment
SK T-Tower is the headquarters of SK Telecom. Inside the building, different types of mobile devices, such as laptops, smartphones and tablets, are in use, and with the increase in WLAN traffic and the use of quality multimedia data, the volume of wireless data sees an explosive growth. Users want limitless Internet access in various places in addition to designated areas.