Company Size
1,000+
Country
- Worldwide
Product
- Anodot
- Snowflake
Tech Stack
- AI/ML
- Data Warehousing
- Real-time Analytics
Implementation Scale
- Enterprise-wide Deployment
Impact Metrics
- Customer Satisfaction
- Productivity Improvements
- Revenue Growth
Technology Category
- Analytics & Modeling - Machine Learning
- Analytics & Modeling - Real Time Analytics
- Platform as a Service (PaaS) - Data Management Platforms
Applicable Functions
- Business Operation
- Sales & Marketing
Use Cases
- Predictive Maintenance
- Real-Time Location System (RTLS)
- Supply Chain Visibility
Services
- Data Science Services
About The Customer
The customers of Anodot are companies that generate a large volume of data and require real-time monitoring and analysis. These companies span various industries such as fintech, ecommerce, gaming, adtech, and streaming. They have millions of customers across the globe and must manage millions of daily business metrics involving product usage, application performance, APIs, log-ins, and payment gateways, among others. These companies use Snowflake warehouse for their data storage and management needs. They require a solution that can handle the volume and complexity of their data, provide real-time alerts on mission-critical anomalies, and reduce the time and cost of incident resolution.
The Challenge
Companies are generating more data than ever before, and traditional dashboards are unable to keep up with the volume and complexity of the vital business data collected. This is particularly true for companies using a Snowflake warehouse. The businesses served by Anodot have millions of customers across the globe and must manage millions of daily business metrics involving product usage, application performance, APIs, log-ins, and payment gateways, among others. Traditional manual business monitoring solutions cause significant delays of at least 24 hours or longer in detecting and resolving critical incidents, which threaten to impact customer satisfaction, brand equity, and the company’s bottom line. Transactional and customer experience data is too volatile for static monitoring. Since business data is complex and dynamic, AI/ML-based autonomous solutions are critical for achieving business outcomes and avoiding blind spots. Static monitoring approaches based on dashboards, and manual thresholds aren’t sensitive, robust, or agile enough to withstand this challenge. AI-based early detection of revenue issues and business system failures is nonnegotiable.
The Solution
Anodot’s AI-driven platform uses patented technology to learn the normal behavior of business metrics. It can automatically detect any deviations with zero configuration burden or margin for error. Anodot provides companies using Snowflake the tools to detect and diagnose issues early, resolve them quickly, and take preemptive actions before they turn into crises. This is in stark contrast to the standard BI scenario of using dashboards and manual investigation that can’t keep up with vast data sets. Anodot’s patented correlation engine correlates anomalies across the business for holistic root cause analysis and the fastest time to resolution, leading to significantly improved availability and customer experience relative to traditional dashboards. Anodot is entirely autonomous, which is critical when dealing with large-scale Snowflake datasets as there’s no need to define what data to look for or when and no manual thresholds to set up or update. When the business needs change, operators create new use cases on the fly across the entire spectrum of Snowflake warehouse data.
Operational Impact
Quantitative Benefit
Case Study missing?
Start adding your own!
Register with your work email and create a new case study profile for your business.
Related Case Studies.
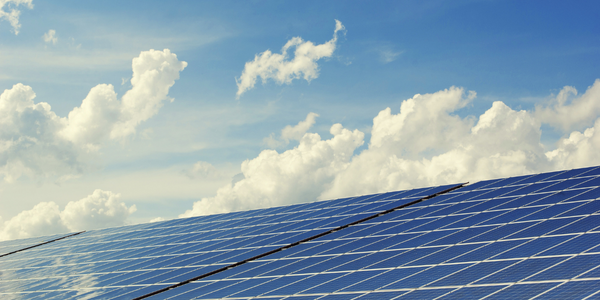
Case Study
Remote Monitoring & Predictive Maintenance App for a Solar Energy System
The maintenance & tracking of various modules was an overhead for the customer due to the huge labor costs involved. Being an advanced solar solutions provider, they wanted to ensure early detection of issues and provide the best-in-class customer experience. Hence they wanted to automate the whole process.
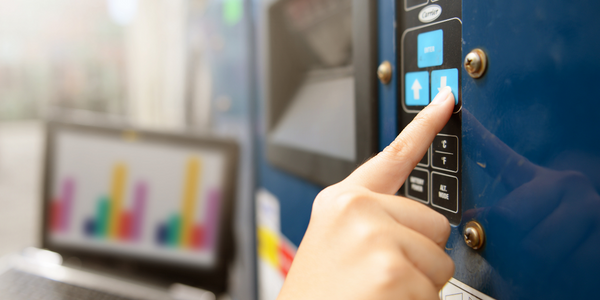
Case Study
Remote Temperature Monitoring of Perishable Goods Saves Money
RMONI was facing temperature monitoring challenges in a cold chain business. A cold chain must be established and maintained to ensure goods have been properly refrigerated during every step of the process, making temperature monitoring a critical business function. Manual registration practice can be very costly, labor intensive and prone to mistakes.
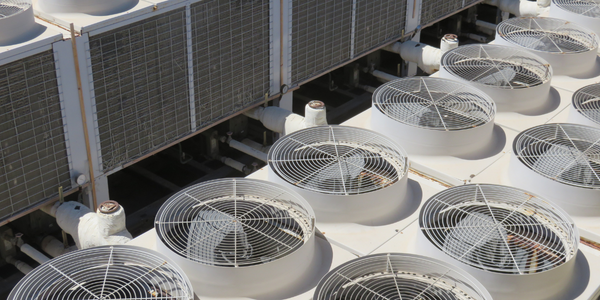
Case Study
Predictive Maintenance for Industrial Chillers
For global leaders in the industrial chiller manufacturing, reliability of the entire production process is of the utmost importance. Chillers are refrigeration systems that produce ice water to provide cooling for a process or industrial application. One of those leaders sought a way to respond to asset performance issues, even before they occur. The intelligence to guarantee maximum reliability of cooling devices is embedded (pre-alarming). A pre-alarming phase means that the cooling device still works, but symptoms may appear, telling manufacturers that a failure is likely to occur in the near future. Chillers who are not internet connected at that moment, provide little insight in this pre-alarming phase.
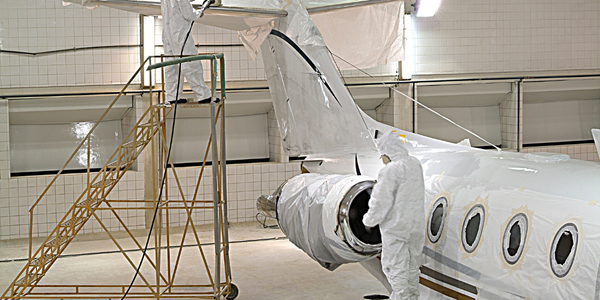
Case Study
Aircraft Predictive Maintenance and Workflow Optimization
First, aircraft manufacturer have trouble monitoring the health of aircraft systems with health prognostics and deliver predictive maintenance insights. Second, aircraft manufacturer wants a solution that can provide an in-context advisory and align job assignments to match technician experience and expertise.
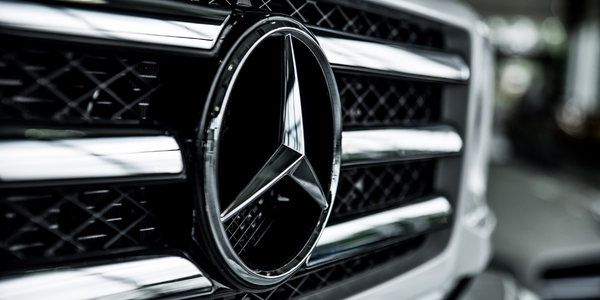
Case Study
Integral Plant Maintenance
Mercedes-Benz and his partner GAZ chose Siemens to be its maintenance partner at a new engine plant in Yaroslavl, Russia. The new plant offers a capacity to manufacture diesel engines for the Russian market, for locally produced Sprinter Classic. In addition to engines for the local market, the Yaroslavl plant will also produce spare parts. Mercedes-Benz Russia and his partner needed a service partner in order to ensure the operation of these lines in a maintenance partnership arrangement. The challenges included coordinating the entire maintenance management operation, in particular inspections, corrective and predictive maintenance activities, and the optimizing spare parts management. Siemens developed a customized maintenance solution that includes all electronic and mechanical maintenance activities (Integral Plant Maintenance).
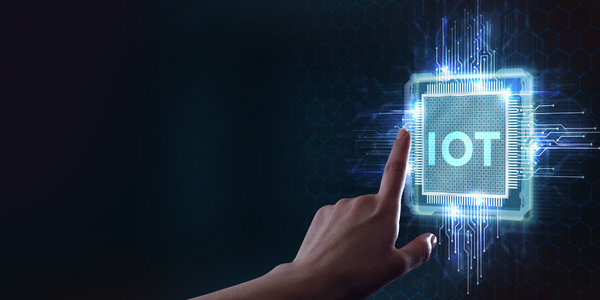
Case Study
Leading Tools Manufacturer Transforms Operations with IoT
Stanley Black & Decker required transparency of real-time overall equipment effectiveness and line productivity to reduce production line change over time.The goal was to to improve production to schedule, reduce actual labor costs and understanding the effects of shift changes and resource shifts from line to line.