Product
- Pachyderm
Tech Stack
- Python
- Docker
- Kubernetes
Implementation Scale
- Enterprise-wide Deployment
Impact Metrics
- Innovation Output
- Productivity Improvements
Technology Category
- Analytics & Modeling - Machine Learning
- Platform as a Service (PaaS) - Data Management Platforms
Applicable Industries
- Agriculture
Applicable Functions
- Product Research & Development
- Quality Assurance
Use Cases
- Machine Condition Monitoring
- Predictive Maintenance
Services
- Data Science Services
- System Integration
About The Customer
Epona Science is a company that specializes in the horse racing industry. They focus on buying, breeding, and identifying the best racehorses in the world. With every thoroughbred being a multi-million dollar investment, the stakes are high. Epona Science aims to revolutionize the traditional horse racing industry by using machine learning, statistical analysis, and science. They believe that factors such as the horse's entire genetic profile and lineage, its height and gait, and even the size of its heart can make a significant difference in its performance. Epona Science is committed to providing buyers and breeders with the best information possible to give them an edge in picking the next legendary champion.
The Challenge
Epona Science is a company that specializes in buying, breeding, and identifying the best racehorses in the world. The racehorse business is a traditional industry where buyers often rely on pedigree or trusted breeders' instincts to choose horses. However, Epona Science believes that these are not the best predictors of success. They aim to revolutionize the industry by using machine learning, statistical analysis, and science. They have discovered that factors such as the horse's entire genetic profile and lineage, its height and gait, and even the size of its heart can make a significant difference in its performance. However, gathering all this data, cleaning it, standardizing it, and getting it into a consistent format that their machine learning models can train on is a significant challenge. The data comes from various sources worldwide, including x-rays, genetic profiles, and track records from previous races.
The Solution
Epona Science chose Pachyderm to help them deal with their data challenges. Pachyderm stood out for its ability to handle everything from data lineage, data transformation and versioning, to containerization. The platform's versioning and provenance tools allow Epona's team to roll backwards and forwards, looking at what changed, when, and why. This is crucial for their sensitive models, as they often need to conduct a detailed forensic analysis to figure out where the model went wrong so they can fix it quickly. Pachyderm also offers more flexibility than alternatives like Airflow, which are more rigid and not designed for Kubernetes first. The platform allows Epona to easily string together a series of independent and isolated tools into a smooth pipeline. This has changed the way they do business, as they had to run everything in isolation in the past. Now, their model development throughput is effectively continuous. Every single model they have and every sample, especially genetics samples, runs through the pipeline, gets tested, and uploaded to the website in minutes.
Operational Impact
Quantitative Benefit
Case Study missing?
Start adding your own!
Register with your work email and create a new case study profile for your business.
Related Case Studies.
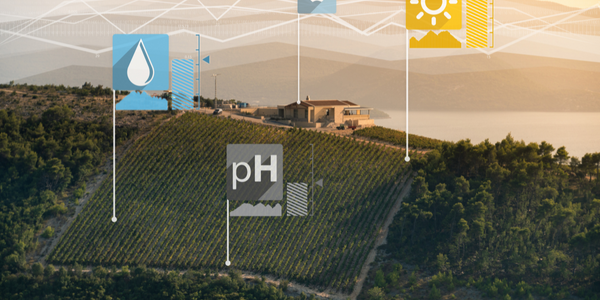
Case Study
Intelligent Farming with ThingWorx Analytics
Z Farms was facing three challenges: costly irrigation systems with water as a limited resource, narrow optimal ranges of soil moisture for growth with difficult maintenance and farm operators could not simply turn on irrigation systems like a faucet.
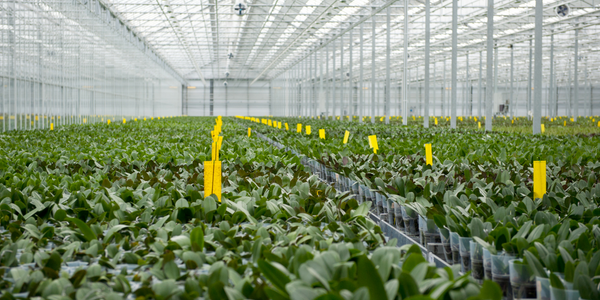
Case Study
Greenhouse Intelligent Monitoring and Control Solution
Farming Orchids is the most successful form of precision farming in Taiwan, and also the most exported flower. Orchids need a specific temperature and humidity conditions to grow and bloom, and its flowering time may not be in line with market demands, so the price collapses when there is overproduction. Therefore, some farmers began to import automated greenhouse control systems for breeding and forcing, which not only improves quality, but also effectively controls the production period and yield to ensure revenue. In 2012, an orchid farmer built a Forcing Greenhouse of about 200 pings (approximately 661 Square Meters) in Tainan, Taiwan. The system integrator adopted Advantech’s APAX-5000 series programmable automation controllers to build the control platform, coupled with Advantech WebAccess HMI/SCADA software, to achieve cloud monitoring. The staff of the orchid field can monitor important data anytime via smart phone, iPad, and other handheld devices, and control the growth and flowering conditions. System requirements: In the past, most environmental control systems of orchid greenhouses in Taiwan used PLCs (Programmable Logic Controller) with poorscalability and control, and could not be connected to the Internet formonitoring from the cloud. For advanced database analysis and networking capability, the PC platform must be adopted. Therefore, PAC Systems (Programmable Automation Controller) with both PLC programming capabilities andPC functions is a better choice.The environmental control of the Orchid greenhouse switches on and off devices like fan, shade net, cooling/heat pump, liquid flow control, water-cooling wall etc. It is controlled by a control panel of electric controllers, and is driven by a motor, to adjust the greenhouse temperature, humidity, and other environmental conditions to the set parameters.
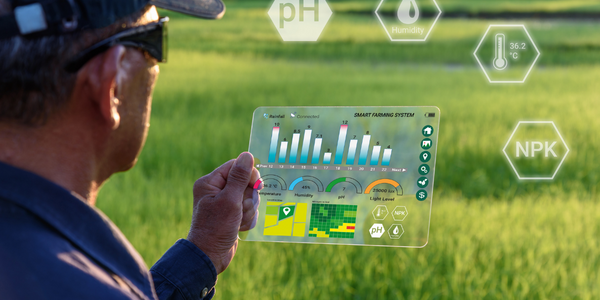
Case Study
Enabling Internet of Things Innovation in Agriculture
DigiBale, wanted to apply technology know-how and IP from implementations successfully to more agriculture sectors including cotton, forestry, sugarcane and cattle. However, farmers and growers still have worries about the connected technology.
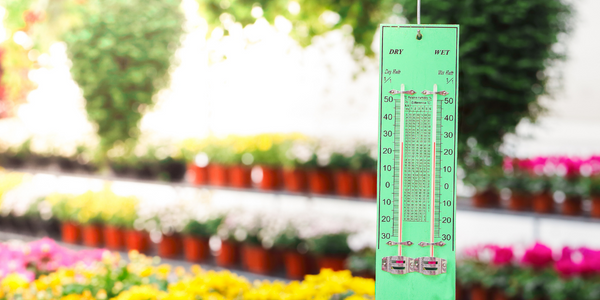
Case Study
Precision beekeeping with wireless temperature monitoring
Honeybees are insects of large economic value and provide a vital service to agriculture by pollinating a variety of crops. In addition, bees provide us with valuable products such as honey, beeswax, propolis, bee venom, etc. Monitoring of honeybee colony health, population, productivity, and environmental conditions affecting the colony health have always been exceedingly difficult tasks in apiculture. Research has shown that even small deviations (by more than 2°C) from the optimal temperatures have a significant influence on the development of the brood and the health of adult bees.