Customer Company Size
Large Corporate
Product
- Gathr
Tech Stack
- Jira
- Jenkins
Implementation Scale
- Enterprise-wide Deployment
Impact Metrics
- Customer Satisfaction
- Productivity Improvements
Technology Category
- Analytics & Modeling - Real Time Analytics
Applicable Functions
- Business Operation
Use Cases
- Predictive Quality Analytics
- Root Cause Analysis & Diagnosis
Services
- Data Science Services
About The Customer
The customer is a leading media and entertainment company with a presence in over 150 countries. The company has a headcount of over 3000 employees. In addition to its newly launched subscription-based streaming application, the company has other apps that require frequent updates. The company's internal DevOps team manages these applications. However, the company was seeking to improve visibility into performance, identify areas for improvement, and gauge customer experience.
The Challenge
The company, a leading media and entertainment entity with a presence in over 150 countries, was facing challenges in managing its applications, including a newly launched subscription-based streaming application. The company's internal DevOps team was responsible for managing these applications, but the company wanted to improve visibility into performance, identify areas for improvement, and gauge customer experience. However, they lacked a standard framework to measure DevOps success and relied on monthly manual reports to understand the team's health and performance. This approach had limitations in analyzing DevOps data and metrics. Furthermore, frequent bugs and a longer time to resolve issues led to a poor customer experience.
The Solution
The company partnered with Gathr to implement DORA metrics, a widely accepted standard for tracking DevOps success. The solution included a unified dashboard to monitor the metrics, enabling the company to understand the root cause of issues and drive decisions for continuous performance improvements. The solution featured out-of-the-box connectors to unify data across tools like Jira and Jenkins, providing end-to-end visibility across development, deployment, and operations. The visual dashboard monitored the four key DORA metrics (Deployment Frequency, Lead Time for Changes, Change Failure Rate, and Time to Restore Service). The solution also allowed for custom metrics to meet evolving requirements for strategic and operational decision making and trend analysis to gauge metrics over a defined period and analyze Lead Time based on parameters like Assignee, Type, Priority, etc.
Operational Impact
Quantitative Benefit
Case Study missing?
Start adding your own!
Register with your work email and create a new case study profile for your business.
Related Case Studies.
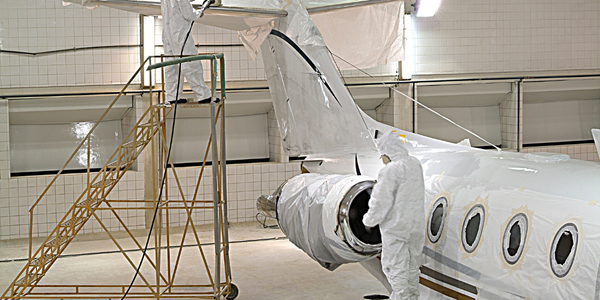
Case Study
Aircraft Predictive Maintenance and Workflow Optimization
First, aircraft manufacturer have trouble monitoring the health of aircraft systems with health prognostics and deliver predictive maintenance insights. Second, aircraft manufacturer wants a solution that can provide an in-context advisory and align job assignments to match technician experience and expertise.
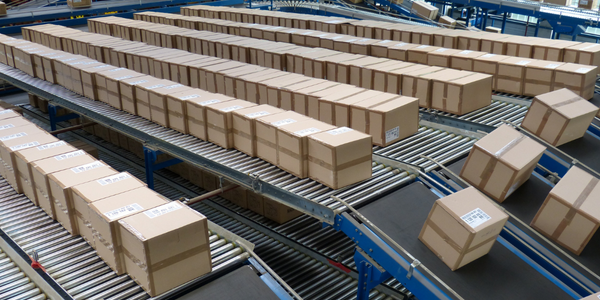
Case Study
IoT Data Analytics Case Study - Packaging Films Manufacturer
The company manufactures packaging films on made to order or configure to order basis. Every order has a different set of requirements from the product characteristics perspective and hence requires machine’s settings to be adjusted accordingly. If the film quality does not meet the required standards, the degraded quality impacts customer delivery causes customer dissatisfaction and results in lower margins. The biggest challenge was to identify the real root cause and devise a remedy for that.
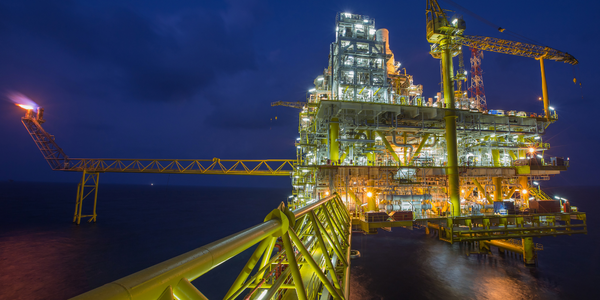
Case Study
Large Oil Producer Leverages Advanced Analytics Platform
Approximately 17,000 wells in the customer's portfolio have beam pump artificial lift technology. While beam pump technology is relatively inexpensive compared to other artificial lift technology, beam pumps fail frequently, at rates ranging from 66% to 95% per year. Unexpected failures result in weeks of lost production, emergency maintenance expenses, and costly equipment replacements.
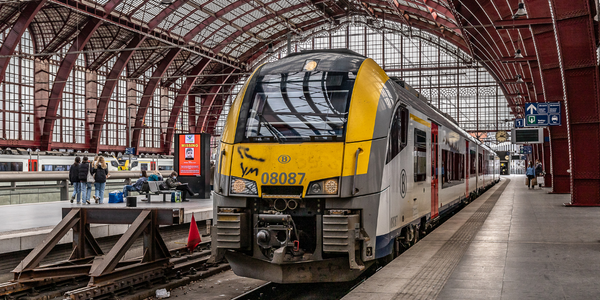
Case Study
The Internet of Trains
Train operators the world over are expected to work miracles, i.e. never to be late. So, with acute service and availability targets to meet, an efficient maintenance program is important. And data-enabled functionality is a must for Siemens. Reactive maintenance (after an incident) and routine, preventive maintenance with its visual inspections and scheduled exchange of components, are no longer enough. We’ve moved on to more cost-effective, condition-based, predictive maintenance. The actual condition of components is measured via the transfer and remote monitoring of diagnostic sensor data; data which is also used to analyse patterns and trends. This helps predict when a component is likely to fail, so it can be repaired before anything untoward happens. To ensure the commercial sustainability of this approach, Siemens needs to use and re-use existing data, creating a kind of ‘Internet of Trains’. Towards this end, they’re analysing sensor data in near real time, which means they can react very quickly, ensuring that customer transport services aren’t interrupted. “It is really difficult to define every issue before it impacts operations using only data from the trains”, Kress explains. However, recent success stories prove that everything is possible.
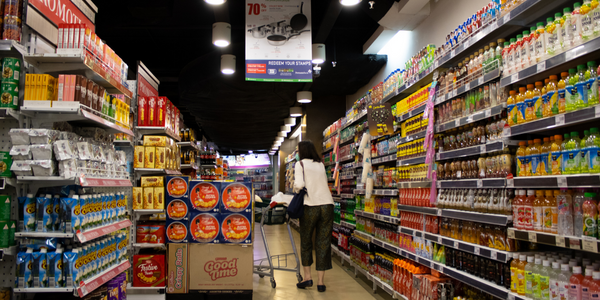
Case Study
FMCG Case Study – CPG Line Monitoring
The leading CPG company operates several warehouses, mostly closer to the last distribution point (Large retailers). Products in various categories are packaged in specific delivery or display cartons at these facilities. As most CPG businesses consist of high volume with low margins, optimizing every operation and effective utilization of resources add up to profit margins. The key problem at these warehouses is the lack of visibility into reasons for machine breakdowns or idle time, thereby delay in delivery. The sunrise meetings lead to post-mortem of delivery issues like delay or quality. The customer wanted to implement real-time line monitoring and alert system to gain control over downtime issues and implement improvement measures.

Case Study
Prevent Process Inefficiencies with Automated Root Cause Analysis
Manufacturers mostly rely on on-site expert knowledge for root cause analysis. When the defective product is sent to lab for analysis, it is laborious and always a post-mortem one. Manufacturers that collect data from IT and OT also need a comprehensive understanding of a variety of professionals to make sense of it. This is not only time consuming, but also inefficiencient.